AI Presents Cybersecurity Challenges, Opportunities
AI tools are being used for prompt engineering jailbreaks, malware coding and more efficient phishing campaigns
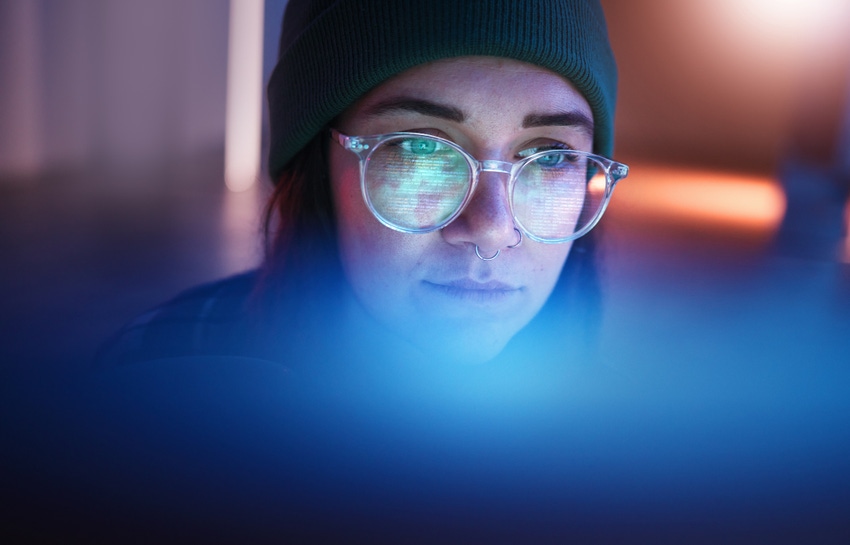
Advancements in AI technology are rapidly accelerating. For example, the differences between the first iteration of Open AI’s original ChatGPT in 2018 and today’s GPT-4 are monumental.
The same development speed applies to cybersecurity and the use of AI in operational and defensive environments.
The effectiveness and safety of large language models (LLMs), particularly in critical fields like cybersecurity, depend heavily on the integrity and quality of their training data. Persistent attempts by malicious actors to introduce false information pose significant challenges, potentially compromising the model’s outputs and, by extension, the security postures of those relying on these tools for information and guidance.
This underscores the importance of continuous monitoring, updating and curating sources used in training LLMs. Developing robust mechanisms to detect and mitigate the influence of incorrect information is key.
In security, AI is being integrated into security orchestration, automation and response (SOAR) products for straightforward tasks like modifying firewall rules or managing IP addresses and enhancing response capabilities.
Offensive Applications
From previous breaches from hashes via source code and customer information to armies of hackers that share information, there is an abundance of breached data and open-sourced information online.
This means there is a good chance that AI can and will be used to change small things in previously used breaches to bypass security, based on signature detection, for example.
The most prominent ways in which threat actors are currently using generative AI tools include:
Prompt engineering jailbreaks. Prompts like “Do anything now” are intended to bypass the core behavioral prompts applied by the owner of the generative AI tools, for example, the limitations placed by OpenAI on ChatGPT.
Malware coding. In the same way that software engineers are using generative AI tools to speed up coding tasks, malware writers, particularly “script kiddies,” are using these tools to accelerate their capabilities.
More effective phishing and social engineering campaigns. Generative AI tools are being used to write more convincing phishing emails, free of typical grammatical errors.
There are also privacy concerns. Different tools may have different levels of privacy and incorrect usage may result in data leakage. One example is that the public, free version of ChatGPT uses data input from prompts for further training of the model, whereas the paid version provides an option to exclude your data from being used for training. Samsung made headlines when it banned the use of tools like ChatGPT after discovering staff had uploaded sensitive code to the platform.
Shadow AI usage could become a problem, too. Similarly to the principle of shadow IT, where multiple business units or individuals within an organization would purchase and use cloud services without the knowledge of the IT team, there is a concern that users may behave similarly with the use of AI.
Defensive Applications
When implementing AI and LLMs to enhance cybersecurity measures, it's essential to clearly define the primary motivations for integrating these technologies and start by identifying the specific challenges that AI is intended to address.
For example, are you trying to reduce alert fatigue, where security teams are overwhelmed by a high volume of notifications? Perhaps the issue lies with the speed of your current security information and event management (SIEM) systems, which may rely on slower SQL databases that take a longer time to process and produce results.
Alternatively, consider if there are complex processes that are prone to human error, such as joiner-mover-leaver (JML) protocols, which could be streamlined and made more reliable through automation. Understanding and clearly articulating the precise function of AI in your cybersecurity strategy is crucial for targeting its deployment effectively and ensuring it addresses the right issues. It is still based on data and fine-tuning.
When you set up AI-driven defensive capabilities in cybersecurity, the effectiveness of these systems fundamentally relies on the data they receive. This data, often referred to as telemetry, includes logs, metrics, events and other signals from across your IT environment. The AI uses this telemetry to make informed decisions and establish rules for identifying and responding to potential security threats.
For instance, if your AI system is tasked with detecting unauthorized access, it will analyze telemetry data such as login attempts, access logs and network activity. Based on this data, the AI can learn patterns of normal behavior and detect anomalies that may signify a security breach. Essentially, the quality and scope of the input data directly influence the AI's ability to secure an environment effectively. The AI models depend on this data to build accurate and reliable rules and decision-making processes that help protect against cyber threats.
Today, multiple vendors are using AI and LLMs to make faster decisions based on trends for product development, feature enrichment and utilization. The benefit is faster decisions based on reliable, clean data with no subjectivity as it is evidence-based.
Dynamic watchlists for activity and devices
This leverages AI to monitor and evaluate activities and devices in real time, identifying potential threats based on historical data and evolving patterns. It dynamically adjusts watchlists, ensuring the system focuses on the most relevant threats at any given time. This adaptability reduces the chances of overlooking emerging threats and decreases false positives, making security monitoring more efficient.
Custom rule functionality based on data
AI and LLMs enable sophisticated, data-driven rules for cybersecurity systems to be created. These rules can adapt to changing threat landscapes by learning from new data, allowing for a more responsive, proactive security posture. Custom rules crafted from deep insights into an organization’s specific data environment can help pinpoint unusual or malicious activities.
Minor variations in signature-based detection
Traditional signature-based detection methods can be rigid, failing to catch slightly modified malware. AI enhances these methods by identifying minor variations in known malware signatures, offering the ability to catch modified threats that might otherwise slip through. This application of AI makes signature-based detection more robust and less susceptible to evasion techniques.
Tailor-made threat intelligence
By combining external threat intelligence with an organization’s specific telemetry data, AI can develop customized threat intelligence, applicable to the unique security concerns of the company. This tailor-made approach ensures defensive strategies are highly relevant and effective, focusing on protecting against the most pertinent threats.
Big data analytics
The vast amount of data modern enterprises generate can be overwhelming for traditional security tools. But AI excels in analyzing big data, extracting meaningful patterns and identifying anomalies that might indicate a security threat. This enables organizations to leverage their data more effectively in defending against attacks.
Deep behavioral analytics
Deep behavioral analytics go beyond simple pattern recognition, analyzing the nuanced behaviors of users and systems to identify malicious activity that might not trigger traditional detection mechanisms. By understanding the baseline of normal behavior, AI-driven systems can detect deviations that suggest a compromise or attack, often before the damage is done.
Generative AI and its applications are still in their infancy. We are barely scratching the surface of the possibilities. The strides made between GPT-1 and GPT-4 are huge and it is exciting for the future.
How we govern these AI tools is going to be paramount for both countries and organizations. But until they can discern emotional responses, the threat is purely mathematical.
AI-generated threats are growing and they will not stop. Just make sure if you bring AI in, it is for the right reasons and that is locked down as tight as it can be.
This article first appeared in IoT World Today's sister publication AI Business.
About the Author(s)
You May Also Like