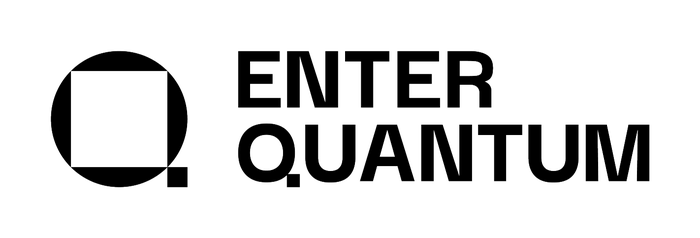
Connects decision-makers and solutions creators to what's next in quantum computing
Quantum Vs. Classical AI Computing: Expert Reactions
Report suggests AI’s ability to simulate quantum systems poses questions about quantum’s long-term commercial viability
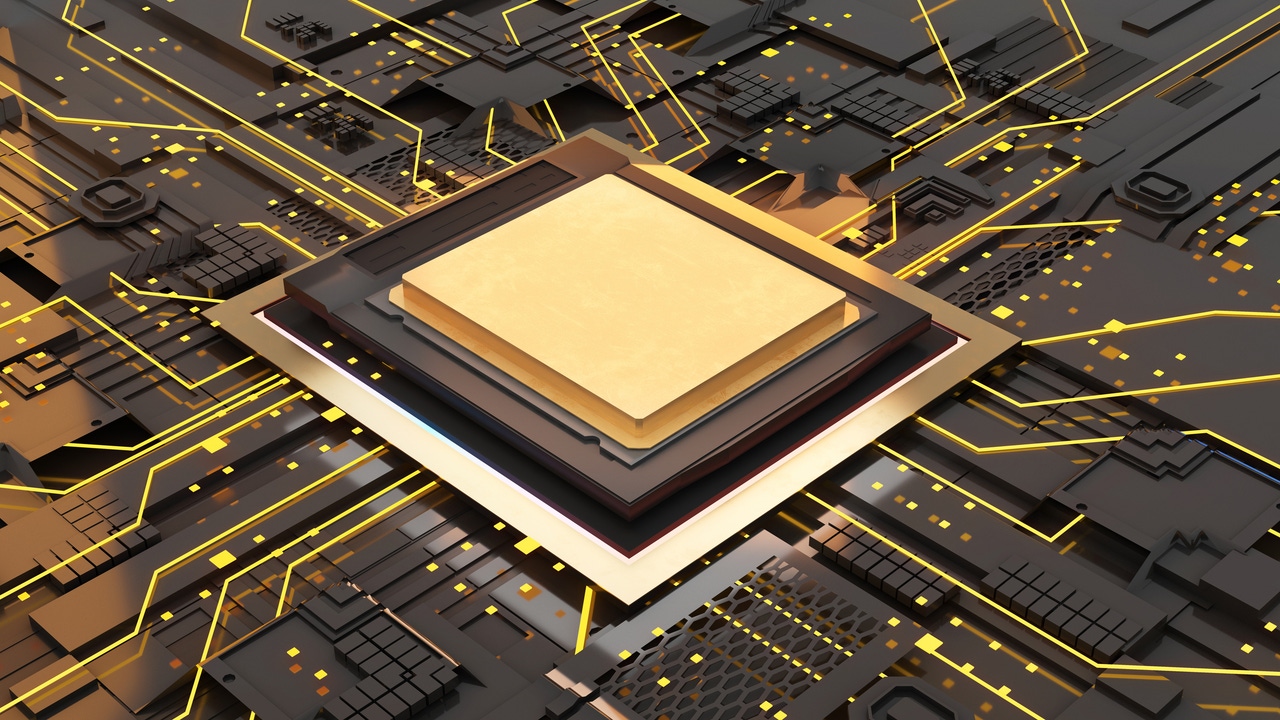
One of the arguments in favor of quantum computing over classical technology is that it naturally excels at modeling quantum behavior, such as creating novel molecules or the reaction of the human body to drugs. This has led to it being hailed as the future for applications including drug development and novel materials for the sustainable energy ecosystem.
However, recent reports, including a viral article from MIT Technology Review, have cast doubt on this often touted benefit. They suggest AI running on classical systems can outperform quantum computing at modeling physics and chemistry, at least in the shorter term.
The argument is nuanced by evidence that quantum computing could accelerate AI systems and bring down its substantial and growing energy demand.
Quantum and AI experts have weighed in to examine the truth behind the claims.
Yuval Boger, chief commercial officer, QuEra Computing
A hybrid quantum-classical approach could be the most effective future direction
While AI has indeed shifted expectations, quantum computing still holds unique potential in areas AI struggles to reach. How soon quantum computing will have a transformative commercial role remains to be seen, but it has significant value as a tool for pushing the boundaries of fundamental science. In the short term, however, AI’s cost-effectiveness and capability make it a more practical choice for many industries, placing commercial pressure on quantum computing to deliver on its long-term promises.
AI can model certain quantum properties with high accuracy, reducing the need for quantum computers in some applications. However, there are strongly correlated systems, e.g., high-temperature superconductors, where classical and AI models still struggle. Quantum computers theoretically have an advantage here, especially once they become fault-tolerant and scalable, but that hardware is still in development.
Quantum computers operate under the same rules that govern quantum systems, potentially enabling them to simulate strongly correlated interactions more naturally than classical computers can. However, this is currently more of a theoretical advantage; only when quantum hardware scales significantly will we know if this promise holds true across real-world applications.
Many in the field see a hybrid approach as the most effective future direction. Combining classical, AI-driven methods for less complex problems with quantum algorithms for cases where quantum effects are strong could allow researchers to leverage the strengths of both technologies. This approach acknowledges both AI ’s rapid advancements and quantum computing’s potential.
Many experts view quantum computing as a long-term investment in fundamental science rather than a technology with immediate, widespread commercial applications. Quantum computers could eventually unlock deeper insights into fields like statistical mechanics, high-energy physics, or quantum field theory, which are traditionally difficult to study. However, these applications are years, if not decades, from realization.
Another important point is that AI systems need to be trained and quantum computers offer the opportunity to create training data for AI systems by simulating complex molecules, providing data beyond what is available experimentally. This was covered very recently at the Quantum + AI conference in NYC by researchers from Microsoft and others.
Quantum computing is unlikely to “overtake” AI in the traditional sense, as they are fundamentally different technologies with distinct strengths. However, we might consider a future where quantum computing achieves a competitive advantage in specific areas, particularly in solving complex, high-value problems that classical AI struggles with, such as strongly correlated quantum systems or advanced materials science simulations.
In the three- to five-year timeframe, computing will mostly serve experimental and niche purposes. We can expect small-scale demonstrations of quantum advantage in narrowly defined problems, potentially in chemistry and materials science.
Stefan Leichenauer, vice president of engineering, SandboxAQ
Quantum computing will be applied only to the hardest parts of the hardest problems
The MIT Technology Review article summarizes the situation quite well. With the rise of GPUs and AI, classical computing has already proven to be extremely effective in tackling real-world, quantum-native problems, such as those found in drug discovery and materials design. This classical approach will continue to make rapid progress and become more and more capable. That said, there remain some quantum-native problems that are simply too complex for classical computers to solve, regardless of any advances in computational power or algorithmic techniques, AI included. The key question is how many of those ultra-complex problems have practical utility rather than purely academic interest and today, we don’t know the answer.
Quantum computers are still too primitive today to solve most classically intractable problems. The usual example where quantum computers have an exponential advantage is Shor’s algorithm for factoring large numbers, though it will be some years before we have quantum computers capable of executing it at scale. To date, the only classically intractable problems solved on real quantum computers have been artificial ones designed solely for that purpose. Quantum-native problems found in chemistry and physics are the best source of potential future utility for quantum computers, but even in those domains, it will only be the thorniest problems where quantum computers are necessary.
For the foreseeable future, quantum computing will be applied only to the hardest parts of the hardest problems, while all workflows will continue to rely heavily on AI and classical computing. Even as we scale quantum computers, classical AI-driven computing will handle the majority of computational tasks, with quantum computers stepping in only where they’re uniquely suited. We’re still years away from quantum overtaking classical computing, but AI will always play a critical role.
Eleanor ‘Nell’ Watson, IEEE member, AI ethics engineer and AI Faculty, Singularity University
Quantum machine learning promises theoretical speedups for specific machine learning tasks
Artificial intelligence systems, such as reinforcement learning, can now handle complex, non-linear, unbounded problems like protein folding and steering fusion reactors – these capabilities rival the promise of quantum, at a much lower cost and complexity and greater scalability. It's also possible to emulate many of the capabilities of quantum computers within classical ones, side-stepping the need for quantum hardware in some circumstances. Typically, this is prohibitively slow and complicated to do, but for some basic processes, it's fast enough.
Moreover, machine intelligence helps us to optimize various algorithms, which also negates some of the potential value of quantum. For the past 20 years, there has been a constant pattern of a new development in quantum finding a niche application where it's useful, such as in a hedge fund, only for a new algorithmic optimization to render that quantum development moot in a matter of months. AI optimization simply accelerates this iron law.
For near-term practical applications, classical AI may indeed maintain an edge in many domains, particularly where the benefits of quantum computing are offset by technical challenges like error correction and qubit stability. Quantum machine learning (QML) represents an intriguing intersection of these fields, promising theoretical speedups for specific machine learning tasks like quantum neural networks and quantum kernel methods. However, current QML implementations still face major practical challenges, including the overhead of quantum-classical data conversion and the limitations of current quantum hardware. Many proposed QML advantages remain theoretical rather than practically demonstrated.
Gev Balyan, founder and AI Expert, Ucraft
Quantum computing holds unique promise for simulating strongly correlated quantum systems
I believe that, in the short term, classical AI is better suited than quantum computing for many physics and chemistry simulations. Current AI models, particularly neural networks, can already handle large, weakly correlated systems that were initially thought to be prime targets for quantum computing. For instance, AI techniques now rival or even outperform traditional approaches like density functional theory, which has been widely used to simulate molecular structures and properties. In real-world applications like drug discovery and materials science, where time and cost-effectiveness are critical, classical AI is proving highly practical.
However, quantum computing still holds unique promise, especially for simulating strongly correlated quantum systems that AI struggles with. These complex systems, central to future technologies like high-temperature superconductors, are where quantum computing could eventually shine. But with quantum hardware and algorithms still maturing, the gap between AI and quantum solutions remains significant. So, for now, classical AI is the more accessible tool, while quantum computing continues to evolve for more specialized tasks. Ultimately, a hybrid approach may offer the best solution, blending AI’s scalability with quantum’s potential in the most complex simulations.
Cache Merrill, founder, Zibtek
Classical AI outshines as the preferred option because quantum computing is still not fully developed
The cutting edge of quantum computing, undoubtedly, entails insurmountable capacity for exponential problem solving. However, classical AI is that practical workhorse that efficiently performs the modeling and simulations on the outbreak.
Classical AI outshines as the preferred option because quantum computing is still not fully developed, especially in highly complex computational tasks spanning fields such as physics and chemistry. However large language models and their ecosystem tools can handle these scalability issues more effectively than quantum machines, which have yet to demonstrate stability.
Due to this management pillar, all of these obstacles should be handled efficiently without many disturbances, allowing for intricate system modeling without the use of a quantum computer in its rudimentary form, which classical AI’s existing infrastructure affords them. That dependability makes it the ideal candidate for advanced scientific simulations in the near future and beyond.
Thomas Balogun, chartered security professional and cybersecurity expert
Classical AI will remain the more viable option for practical modeling in physics and chemistry
In the debate of classical AI versus quantum computing for modeling physics and chemistry, I believe classical AI has strong advantages in the short term. While quantum computing holds immense promise, it’s still in its early stages, limited by scalability and error rates. Classical AI, on the other hand, has developed rapidly and is already producing meaningful results in complex simulations, often by integrating machine learning with traditional computational methods.
For tasks like molecular modeling, drug discovery and material science, classical AI has shown it can efficiently manage these complex calculations, leveraging high-performance computing resources. By using neural networks and advanced algorithms, classical AI can approximate solutions to complex equations that govern chemical and physical interactions. While quantum computers could theoretically provide exact solutions to these problems, we’re likely years away from a quantum machine that can outpace classical methods in practical applications.
Until quantum hardware and error correction improve, I think classical AI will remain the more viable option for practical modeling in physics and chemistry. In the near term, it offers reliable, scalable solutions that can continue to push forward scientific discovery.
Chad Gerber, founder and chief information officer, Meloscene
We’ll need far more computational power like that promised by quantum computing
This is largely accurate, but only under specific conditions. If we assume we’ve gathered all relevant data in physics and have no major breakthroughs on the horizon, then classical AI can indeed simulate outcomes effectively by running models based on these static data sets. However, this assumption is unlikely to hold.
The reality is that as AI itself advances, it will likely drive further discoveries in physics and other complex fields, generating new data that needs to be integrated and processed. This ongoing influx of information would make classical AI increasingly limited, as it would struggle to handle the exponentially growing data without regurgitating previous findings or facing prohibitive time constraints.
If our understanding of physics keeps expanding, we’ll ultimately need far more computational power like that promised by quantum computing to process the new data efficiently and render genuinely novel insights in a feasible timeframe.
About the Author
You May Also Like