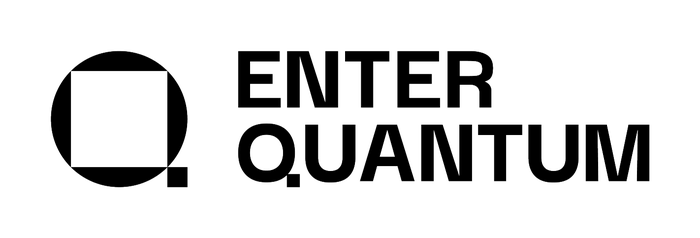
Connects decision-makers and solutions creators to what's next in quantum computing
Google Taps AI to Identify Quantum Errors
Using a quantum simulator, researchers generated hundreds of millions of examples across a variety of settings
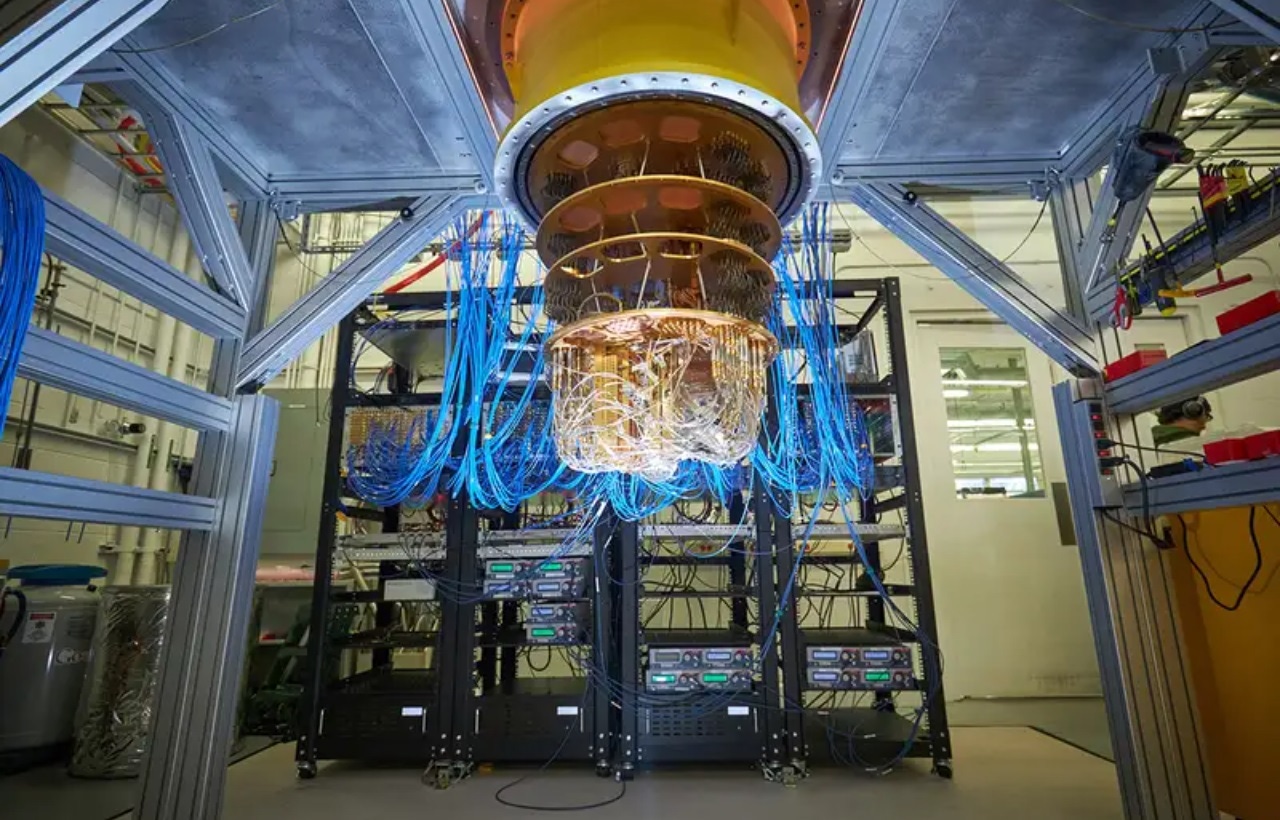
Google’s DeepMind and Quantum AI teams have developed AlphaQubit, a neural network-based decoder that identifies quantum computing errors more accurately than previous methods.
Quantum computers could revolutionize fields such as drug discovery, material design and fundamental physics by solving problems currently intractable for classical computers. However, to scale to a practical size, they need to overcome their susceptibility to noise and errors.
In research published in Nature, AlphaQubit made 6% fewer detection errors than tensor network methods, a slow but highly accurate decoder and 30% fewer errors than correlated matching, an accurate decoder that is fast enough to scale.
The researchers used the Transformer deep-learning architecture to train a model using data from a set of 49 qubits in Google's Sycamore quantum processor.
Using a quantum simulator, they generated hundreds of millions of examples across a variety of settings, error levels and simulations. They then finetuned AlphaQubit for a decoding task by giving it thousands of real-world error samples from a Google Sycamore quantum processor.
To see whether AlphaQubit is scalable, the team trained on simulated quantum systems with up to 241 qubits. It consistently outperformed other decoders, indicating it could scale for larger, future quantum devices.
AlphaQubit is currently too slow for real-time correction in superconducting processors. However, it shows promise for scaling to larger quantum computers and represents an advance toward more reliable quantum computation.
The Google DeepMind and Google Quantum AI team said in a blog post that as quantum computing grows toward the potentially millions of qubits needed for commercially relevant applications, they will need to find more data-efficient ways of training AI-based decoders.
About the Author
You May Also Like