AspenTech Q&A: Why the Democratization of Machine Learning MattersAspenTech Q&A: Why the Democratization of Machine Learning Matters
How machine learning can help bridge the industry’s talent gap.
September 21, 2018
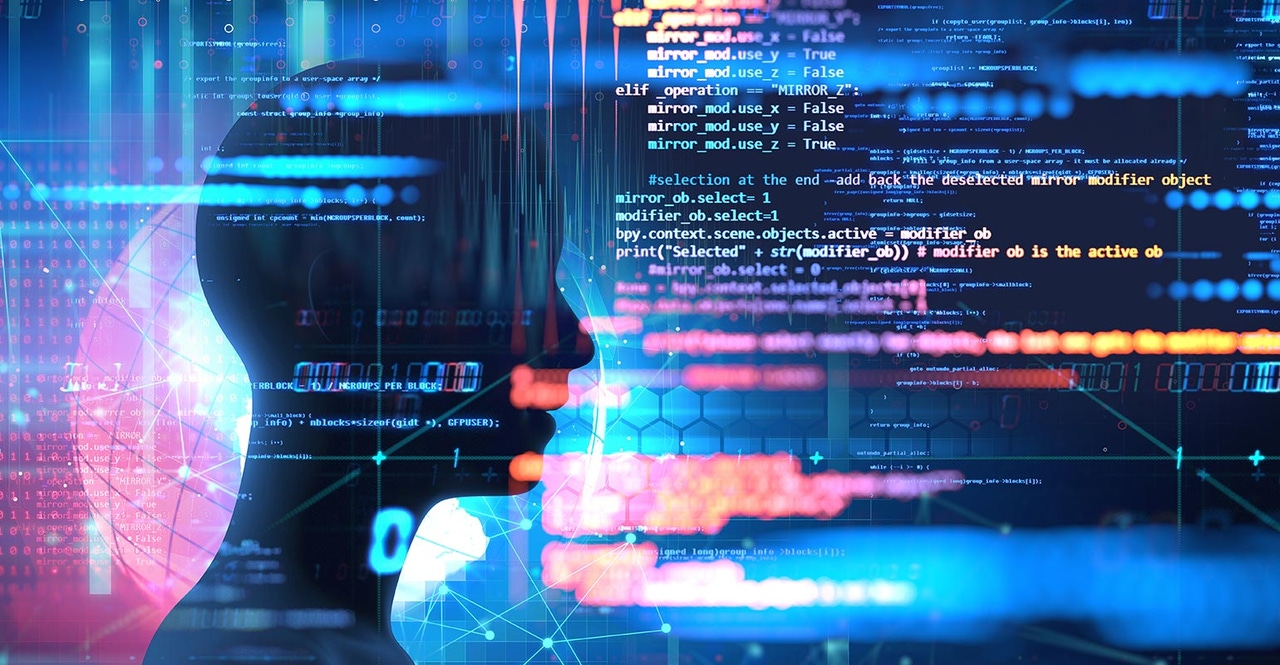
What if your organization had access to the most powerful technological tool every developed, but your workers had no idea how to use it? Or what if you were faced with the Faustian bargain of deploying a technology that promises to dramatically boost your business, but at the cost of having to lay off most of your workers? Those are exaggerated characterizations, but discussions about technologies such as AI and IIoT can often evoke such themes, painting a picture of humans and technology as anything but mutually complementary agents. Yet the fact is, humans define the value of any technology — both literally and figuratively. Too often, companies deploying IIoT, AI and related technologies with the hope of catalyzing business transformation give short shrift to this human element. And thus, AI and IIoT projects can become science projects, or “solutions” without clearly defined problems to solve.
In the following interview, Robert Golightly, senior manager product marketing at AspenTech, takes up such themes. He explains how companies can launch scalable machine learning projects aimed at addressing the human-centered problems that can besiege IIoT deployments and shares information on AspenTech’s approach to use machine learning for industrial applications.
Robert Golightly
What are some examples of use cases that AspenTech is focused on?
Golightly: Every industrial company deals with complex systems in diverse conditions and across many assets. We are in the business of helping companies apply IIoT data — through our machine learning software — to real-world operational excellence. That can be in any number of scenarios, but right now we’re hyperfocused on reliability. It’s a sort of “poster child” use case for IIoT and digital transformation and is proving one of the most fruitful applications of that data.
This can be applied to a variety of scenarios, such as an oil refiner struggling with excessive asset breakdowns, or a chemicals producer with multiple intermediate storage tanks suffering intermittent failures on feed pumps, with each failure resulting in extended downtime and lost product. It could also be an aging and complex electric grid that requires a sophisticated analytics approach — creating a maintenance plan based solely on the average asset lifecycle could result it costly overspend.
AspenTech addresses such use cases to deliver reliability for Industrial companies by using low-touch machine learning and Asset Performance Management (APM) capabilities. AspenTech stands apart from competitors by combining process industry expertise and breadth/depth of mission-critical solutions with decades of experience.
Where does AspenTech see this trend of AI, ML, etc. in terms of empowering workers versus automating certain positions away?
Golightly: According to our recent survey, lack of expertise is a top reason why companies are not adopting data analytics, with more than one-third of companies reporting no in-house data scientists. But, industry is not alone in the fight for top big data talent. It’s competitive against other industries like medical, retail, finance, bio and tech and, as a result, is increasingly turning to technology, like low-touch machine learning, to bridge the gap.
Low-touch machine learning technology basically does the data science for you, requiring minimal human interaction on the data prep and analysis side. It delivers a recommendation or prescription. The worker is very much still needed to take that action or make a final decision, but not to do all the specialized data work. In our use cases, the software provides accurate, advanced notice of impending equipment failures. The machine learning software applies complex, self-learning algorithms to tons of real-time equipment and process data inputs, so process engineers and maintenance teams can make more informed decisions without having to analyze the intricacies of the networks. They can devote their time elsewhere while tedious analytics tasks are automated. Overall, the greatest trend we’ve seen with AI and machine learning empowering workers is the democratization of data science, so everyone can use its capabilities and be successful.
For some companies, IIoT has already helped change how humans work by uniting siloed operations and maintenance teams. How do you see that trend evolving in the next six months to a year?
Golightly: The IIoT is just the connectivity and sensors. It alone cannot change how teams work. But with what low-touch machine learning technology, that does the data analysis and pinpoints problems and breakdowns before they occur, it’s helping unite operations and maintenance teams to come up with the right solutions and move-forward plans. Deployed logically and with appropriate automation, it enables greater agility and flexibility to incorporate current, historical and projected conditions from process sensors and mechanical and process events. Traditional maintenance practices can be improved alongside operations to recognize issues affecting asset degradation.
It’s not just a switch that companies can flip, though. Getting the technology in place is one thing, which more and more are starting to do. Making a cultural change to the ways teams work together around that data is also required, and that’s where this trend is headed next. Already, the newest APM solutions are improving reliability, lifting net product output and increasing profitability. The early adopters are benefiting, and others are quickly jumping on board to stay competitive.
How is AspenTech working to train the next generation of workers to meet the demands of AI-enabled industry in the future?
Golightly: The competency gap is one of the most pressing challenges faced by industry today. It is estimated that close to 20 percent of the current workforce will retire over the next few years — taking decades of skills and experience with them. Therefore, many firms are turning to IIoT and low-touch machine learning to equip millennials with the capabilities needed of experienced professionals or data scientists.
One of the most significant examples is in Operator Training Simulator (OTS) software. OTS simulates real-world conditions to facilitate quick and effective training for new employees. In addition, advanced technology can automate knowledge work to alleviate analytical and administrative strain. For example, machine learning in operations analyzes data to identify when an equipment issue arises and provides real-time guidance on how to address such problems immediately. Instead of having to know everything, all at once, new workers are given clues to help them figure it out.
What is your take on the current data science talent gap?
Golightly: Talent is becoming more important than ever, but how does a chemicals or energy company recruit, develop and retain the talent necessary to keep its business competitive and attract millennials? There are numerous reasons behind why millennials “job hop” so frequently, but one key factor is that many organizations do not give their employees the tools and technology to keep them engaged and motivated.
In the face of the industry’s talent gap, AspenTech is integrating data science capabilities into its technology to meet new workers where they are. Advancements in machine learning empower workers to make complex decisions faster, bridging the gap in expertise. Most prominently, low-touch machine learning is changing the way we work. It helps companies predict where and how an asset breakdown will occur with months — not days — of notice. This notice alleviates sole reliance on data scientists’ expertise and brings operations and maintenance into the equation by giving them the skills needed to make decisions from the data insights provided by autonomous software. By incorporating these capabilities into technology that brings data science and analytics value across all phases of design, operations and maintenance, AspenTech can effectively arm new employees with the expertise they need. In today’s digitized world, a new generation of engineers is pushing the boundaries of what’s possible.
What is your favorite use case?
Golightly: Twenty years ago, if a plant shut down, the unplanned downtime inflicted an immense financial burden on the company. C-level executives of manufacturing and energy firms were making decisions without the power to predict. Like a seismologist tracking the next big earthquake, there was no sure way of knowing when the next shutdown would happen, causing profits to plunge. It was a huge and seemingly intractable problem for industry to address.
At one of customer’s liquified natural gas facility, catastrophic failure of its compressors was occurring regularly and costing upwards of $40 million per occurrence. The facility attempted to identify the cause of these failures but was ultimately unsuccessful. After engaging with AspenTech for a pilot of Aspen Mtell low-touch machine learning, the solution was able to identify the root causes, related to leaks in the heat exchanger that caused mixing of materials. Implementation of Aspen Mtell now provides the facility up to 61 days of notice before a potential failure — crucial to mitigate downtime, reduce costs and enhance safety.
By applying advances in machine learning and the science of maintenance, companies are empowered with technology and real-time operational data that can detect breakdowns before they occur.
What can organizations with machine learning ambitions do to help data scientists and related professionals make the most of their time?
Golightly: Introduction of advanced technology, like machine learning, has revolutionized how data scientists do their work. By leveraging low-touch machine learning to handle the work on the data prep side, data scientists are feed to spend their time analyzing the data to gain valuable insights for business decisions.
About the Author
You May Also Like