Unlocking the True Potential of Mobile AIUnlocking the True Potential of Mobile AI
Performing machine learning and user interaction processes at the edge can put AI in users’ pockets
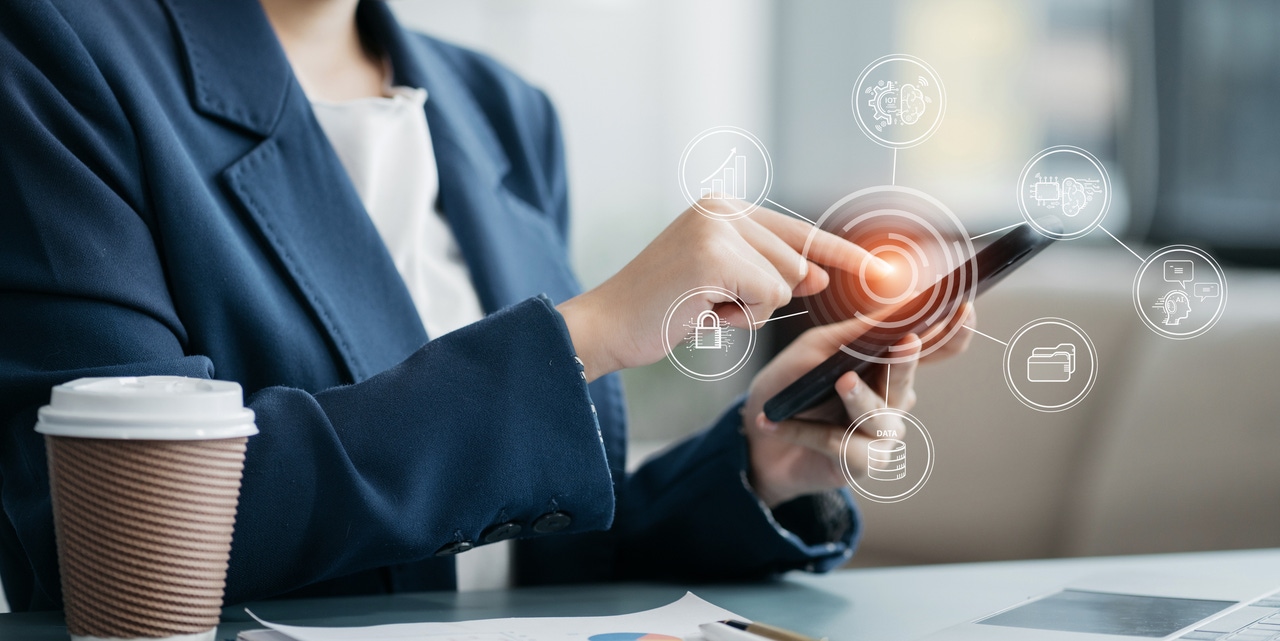
Enterprises are still probing the potential of generative AI and large language models (LLMs). But this doesn’t mean they aren’t already looking at the next frontier. For many, true mobile AI is the holy grail: opening devices to the opportunities traditionally offered by power- and resource-hungry applications.
This isn’t only a fixation on smartphones or other devices – although consumer and business-focused applications will definitely see these as a target. It also means expanding generative AI’s presence across the Internet of Things (IoT), potentially creating truly intelligent operational systems.
The question is how to achieve this. A modern mobile device is a far cry from a Nokia 3210, but even this exponential leap isn’t enough to take advantage of LLMs. So if computational horsepower isn’t the answer, what is?
Building the Right Architecture
The assumption might be that mobile AI can only exist on the cloud with central servers handling the heavy processing and the device just displaying the results. Yet in order to succeed, true mobile AI has to sever the ties that make the cloud indispensable. Connectivity is certainly an issue – an application that is non-functional if it can’t connect to the cloud is immediately less valuable. But there are also the questions of efficiency, speed and data privacy.
The best generative AI applications operate as close to real-time as possible. Every second of delay means data is less up-to-date, responses are slower and every conclusion the AI comes to is less trustworthy. The latency inherent in transmitting data to and from a central server means a cloud-based approach will always fall short. At the same time, organizations need to contend with the bandwidth costs of constant data transmission and the risk of data in transit being compromised. Evidently, the more that can happen on the device itself, the better.
This isn’t to say that cloud servers are irrelevant. Tasks with high computational demands that a mobile device could never match – such as training LLMs and their deep learning models – are still best suited to cloud servers. However, machine learning processes and any task that demands immediate interaction between users and the AI itself need to happen on the device at the edge of the network. This means reducing the computational burden on the device, and ensuring that an architecture is built around the specific demands of edge computing.
Taking the Burden From Devices
Placing the heaviest computing burden on the cloud will help bring true mobile AI within reach. But that burden still needs to be reduced even further before a mobile device can run generative AI applications at the necessary level of performance.
Ultimately, the greatest burden comes from the AI model itself. The more these can be simplified – for instance, reducing the precision of calculations within acceptable parameters – the less the burden on the device. Similarly, optimizing operations elsewhere for greater efficiency will ensure the device can dedicate the most resources possible to its generative AI applications.
The exact approach would depend on each application’s specific needs. But model quantization is generally an essential step towards simplifying the AI model enough to run effectively. Beyond this, approaches such as GPTQ, which compresses models post-training; LoRA, which fine-tunes smaller matrices within a pre-trained model; and QLoRA, which optimizes GPU memory usage for greater efficiency, can further reduce the burden and bring true mobile AI within reach.
Managing Data
Finally, as with any other application, AI demands careful data management. First, data needs to be private and secure. Implementing techniques that preserve privacy, such as preventing data from moving from a device to the LLM’s learning model, and supplementing these with data encryption so that data is protected even if the worst happens, should be a priority.
Second, data needs to be consistent across the whole network. To ensure integrity, data synchronization between edge devices and other cloud or central servers will be critical. With these in place, the organization knows its AI is working off the same data on every device, and so it won’t come up with unexpected or even dangerous conclusions.
In these circumstances, a consolidated data platform that can manage diverse data types, and enable AI models to access local data stores will be a significant advantage. So too will a data platform that enables offline or online access, boosted performance and an enhanced user experience. With the right platform in place, AI applications can operate in a variety of environments, and guarantee responsiveness and reliability, resulting in a far more valuable tool.
The Golden Rule
Ultimately, data management and architecture come back to the golden rule at the heart of many IT strategies: keep it simple. The more organizations can minimize complexity, the more power and focus they can dedicate to AI itself. In a mobile environment, where every single byte of computing horsepower counts, this is critical to success.
This article first appeared on IoT Word Today's sister publication, AI Business.
About the Author
You May Also Like