Research Reveals How ChatGPT Can Help Self-Driving Cars
Purdue University researchers discover the key role large language models play in helping AVs understand passenger commands
September 18, 2024
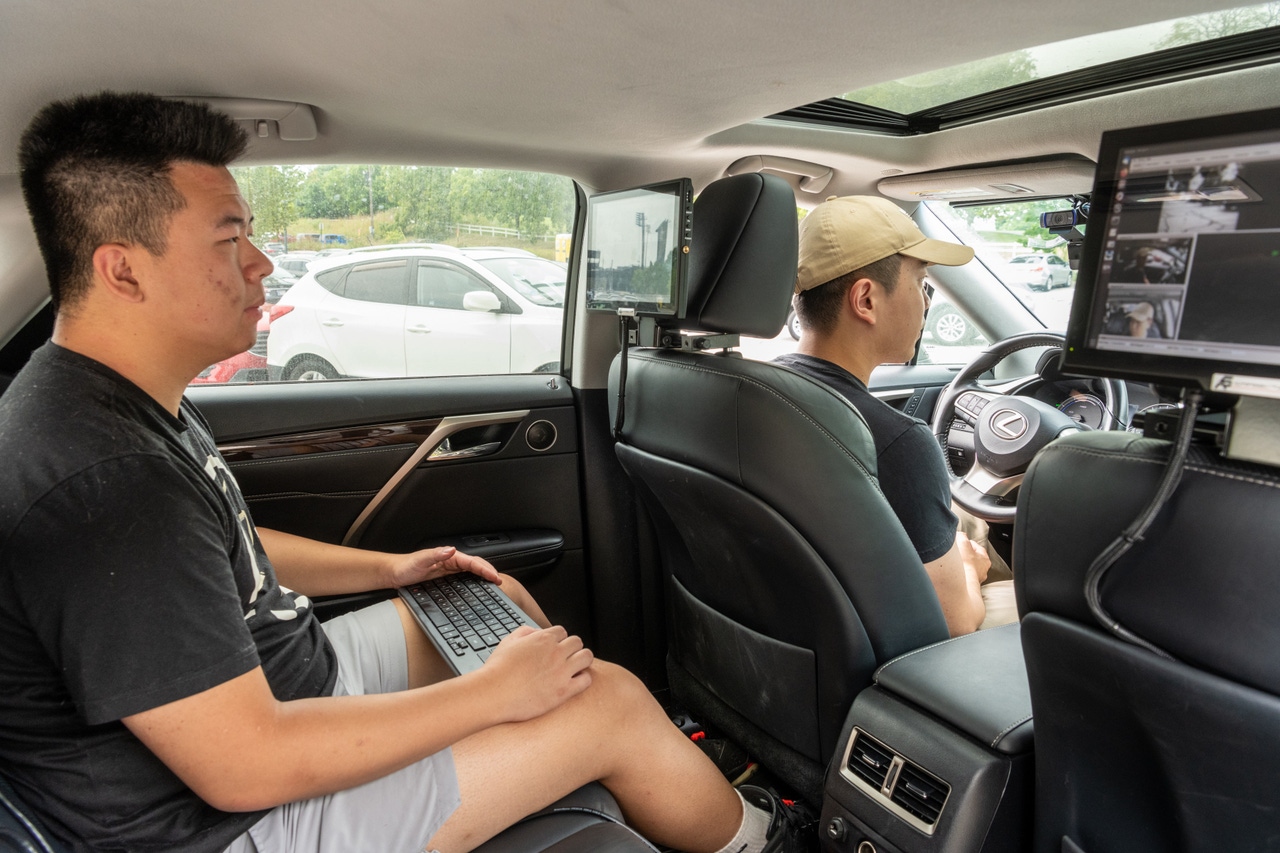
Groundbreaking new research has revealed the extent to which AI-powered chatbots such as ChatGPT could influence autonomous vehicles (AVs) in the future.
A study by engineers at Indiana’s Purdue University has found that large language models (LLMs) could have a key role to play in helping AVs understand the commands of occupants.
And this could even stretch to automatically selecting the optimum route when a comment as simple as “I’m in a hurry,” is used by a passenger.
The research has been led by Ziran Wang, an assistant professor in the Lyles School of Civil and Construction Engineering at Purdue, who said it illustrates how LLMs will facilitate better communication between car and passengers, because they are trained from huge amounts of text data and are constantly learning and evolving.
Wang explained: “The conventional systems in our vehicles have a user interface design where you have to press buttons to convey what you want, or an audio recognition system that requires you to be very explicit when you speak so that your vehicle can understand you.
“But the power of large language models is that they can more naturally understand all kinds of things you say. I don’t think any other existing system can do that.”
In the Purdue study, the LLMs did not drive the AV, but instead assisted the driving through its existing features. Wang and his team discovered that by understanding the occupants better, the AV was able to tailor its driving assistance appropriately.
As preparation for the testing process, Wang and his team trained ChatGPT with explicit prompts, such as “Please drive faster,” to more opaque ones, like “I feel a bit motion sick now.” The LLMs also had various parameters to consider, such as the rules of the road, traffic conditions, weather and information from the car’s sensors.
An AV with Level 4 automation, as defined by the Society of Automotive Engineers, was then given access to these LLMs via the cloud and observed at a proving ground in Columbus, Indiana.
What the Purdue team found was that when the AV’s speech recognition system detected a command from an occupant, the LLM considered the command within the defined parameters, and then generated instructions for the drive-by-wire system – which is connected to the throttle, brakes, gears and steering – on how best to drive.
Other elements of the testing allowed the LLMs to store historical data on a passenger’s preferences, meaning they could generate suitable recommendations when a familiar command was heard.
Feedback from participants was uniformly positive, with occupants expressing a lower rate of discomfort with the decisions that the LLMs-equipped AV made compared to data which shows how people feel when riding in a Level 4 AV that does not have LLM assistance.
However, there is still quite a way to go for the tech. Although the LLMs processed passengers’ commands in an acceptable average time of 1.6 seconds, this would need to be improved when fast responses are required. Wang also pointed out that much more testing needs to be done by automakers, and regulatory approval would be needed to integrate LLMs with an AV’s controls.
The study, called “Personalized Autonomous Driving with Large Language Models: Field Experiments” will be presented on Sept. 25 at the IEEE International Conference on Intelligent Transportation Systems in Edmonton, Canada.
About the Author
You May Also Like