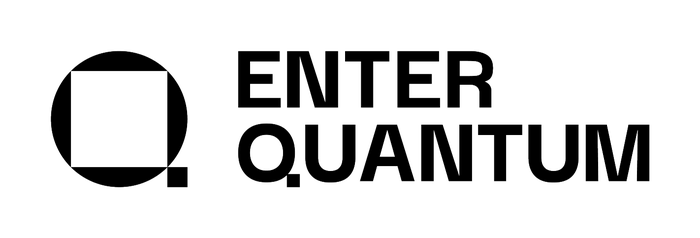
Connects decision-makers and solutions creators to what's next in quantum computing
New Quantum Capabilities Target AI Workloads
Use cases include quantum supply chain optimization, molecular discovery, neural networks
.jpg?width=1280&auto=webp&quality=95&format=jpg&disable=upscale)
D-Wave Quantum has updated its product development roadmap to support bringing new quantum AI applications to market by bringing together quantum optimization, AI and machine learning (ML).
The company plans to make the new capability available on its Leap quantum cloud service to help customers address a variety of AI and ML workloads.
The aim is to support business use cases that require the integration of AI and business optimization, such as quantum supply chain optimization to support AI-predicted product demand requirements.
Quantum computing offers one potential solution to the processing power bottleneck and soaring energy and water demands of data centers supporting the AI boom.
D-Wave’s annealing technology is well suited to solving optimization problems and could support developing better, faster and more energy-efficient AI and ML workloads.
The quantum AI extension to its product development roadmap focuses on three key development areas:
Quantum distributions for generative AI, initially focused on molecular discovery use cases.
Restricted Boltzmann Machine (RBM) architectures, a type of neural network with applications in cybersecurity, drug discovery and high-energy physics data analysis.
GPU integration for the training and support of AI models alongside optimization workloads.
D-Wave also intends to use quantum AI capabilities to build on existing research use cases with its customers. For example:
German researchers used D-Wave’s quantum technology to develop a machine-learning tool that predicts protein-DNA binding with greater accuracy than traditional methods.
Canadian particle accelerator center TRIUMF demonstrated that D-Wave’s quantum technology is faster than classical approaches for simulating high-energy particle-calorimeter interactions. Using AI to create synthetic data could create further efficiencies.
Honda Innovation Lab and Tohoku University developed a method to fine-tune D-Wave’s quantum computers to generate highly accurate samples for training RBMs, which could be more efficient using AI.
“We’re seeing early evidence that annealing quantum computing could play a key role in helping AI/ML with more efficient model training, reduced energy consumption and faster time-to-solution,” said D-Wave CEO Alan Baratz.
“With results demonstrating our annealing quantum computer’s ability to outperform classical techniques, coupled with rapidly increasing demand from our customers for quantum AI solutions that integrate with their business optimization requirements, we believe the impact of D-Wave’s quantum AI solutions could be transformative, bringing a powerful new set of new computing tools for generative AI.”
About the Author
You May Also Like