When Mulling IoT Storage Options, Location Matters
As data streams explode, organizations should be strategic when it comes to IoT storage.
July 24, 2019
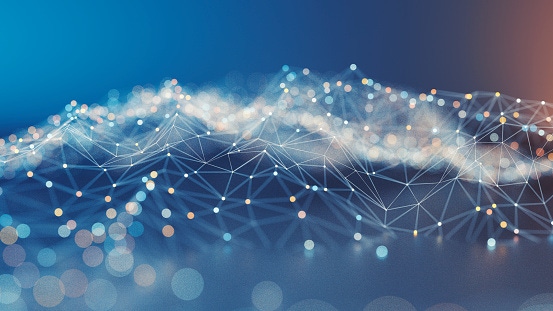
The growing amount of data IoT sensors and machinery generate is forcing companies to rethink their IoT storage strategies.
“Right now, they don’t really know what data is actually useful, and, once analyzed, what business decisions can be helped by that information,” said Dilip Sarangan, global research director, IoT and digital transformation, at Frost & Sullivan.
The 24 billion IoT devices that Gartner estimates will be in use by next year are producing “a ridiculous amount of data that’s just a whole lot of noise right now,” Sarangan said. The question, therefore, is whether to keep it and if so, where to store it.
The push for storage in the cloud, the prime destination for the past several years, is now turning back to on-premises and edge storage, analysts said. And the determination is made application by application — and location by location.
Many organizations with IoT don’t need to go to the cloud, Sarangan said. “They mostly want it on-prem and localized,” he said, especially with latency issues that won’t zero out even in the next decade. “Transmitting data to the cloud doesn’t make sense when most of the applications at the edge need at least a real-time aspect to analysis.”
Eric Burgener, research vice president, infrastructure systems, platforms and technologies, at IDC, agreed that the decision about where data analysis takes place will drive the purchase decision on storage capacity, whether data is stored in the core, or onsite datacenter, in the cloud, or increasingly, at the edge.
Tiered IoT storage options in the cloud, from hot to cold, for example, are less relevant in IoT applications, given the possibility of storing data more locally, according to Burgener. With tiered storage, he said, the basic concept is all data doesn’t have to be stored in the same high-performance, high-cost storage area.
“You only put data there that has to be delivered with high performance and opt for cheaper tiers for data that you don’t need on premises or need access to all that frequently,” he said.
Use cases in which data needs to be analyzed immediately, such as autonomous vehicles, rules out cloud storage. Less time-intensive use cases allow data to be sent back to a central location where machine learning is applied to garner insights.
Certain industries and applications generate significant quantities of data including, utilities, building automation systems (lighting, heating), and surveillance systems. Those surveillance cameras, for example, transmit about 2 megabytes of data per second.
The mix of IoT storage types is attuned to how enterprises want to use it, Burgener said. You might see hundreds of terabytes and tens of petabytes in storage in the data center, but less than a 20-terabyte storage device in an edge location, he said.
Edge devices don’t necessarily need processing power or a ton of storage, just the ability to capture data and send it back to a more centralized location for processing. And depending on where they’re located, those devices need to be ruggedized for weather and have enough power to maintain data until batteries can be changed.
“There’s a real wide continuum of device type so they had to develop different types of storage that can operate with very low power to use in IoT devices,” he said. That tends to be newer solid-state storage technology.”
The data an autonomous vehicle uses to decide whether to stop, for example, is processed at the edge for an instantaneous decision, but once the decision is made, that data is thrown away, Burgener said. Decisions about whether a vehicle needs an oil change based on miles driven, however, is collected at the edge then sent back to a remote location for processing and storage.
IoT has no uniform scenario for storage, said Natalya Yezhkova, research vice president, Infrastructure Systems, Platforms and Technologies, at IDC. “Everything is highly driven by a particular use case,” she said. Some large workloads might be shipped to the cloud for analysis and then returned to an on-premises data center for fine-tuning.
There are also considerations for an organization’s comfort level with cloud storage, the amount of data transfer and how much control over bandwidth they have if large amounts of data need to be moved.
Structured data collected by machinery provides information about characteristics of the machine which have been transferred to data center for analysis. Surveillance videos present huge amounts of unstructured data that may need to be analyzed in real time or often, is never used again.
Also, the amount of storage at the edge is determined by several factors, including how far removed the edge is from the core. Low-power sensors on an oil rig in the ocean, for example, might need additional storage and batter power depending on how often data is uploaded from the device.
“Data can’t just stay idle,” she said, if it is to meet the major goals of IoT: analytics to drive improvements in operations and customer experience.
Most IoT data is unstructured and easy to store in public clouds. And all major cloud providers offer scalable storage systems with little to no charge for data ingress. Cloud also offers big data analysis tools for jobs too big for on-premises datacenters.
When considering storage options, users should make sure data management aligns with workloads and the scale of the application.
“It’s not a settled practice of when to use cloud vs. the datacenter,” Yezhkova said. “It’s almost trial and error for some organizations.”
About the Author
You May Also Like