Why Predictive Maintenance Isn’t a Killer IoT Use Case Yet
Predictive maintenance was supposed to be a defining IoT use case, but IoT-enabled preventative maintenance remains a safer bet for many organizations.
July 11, 2019
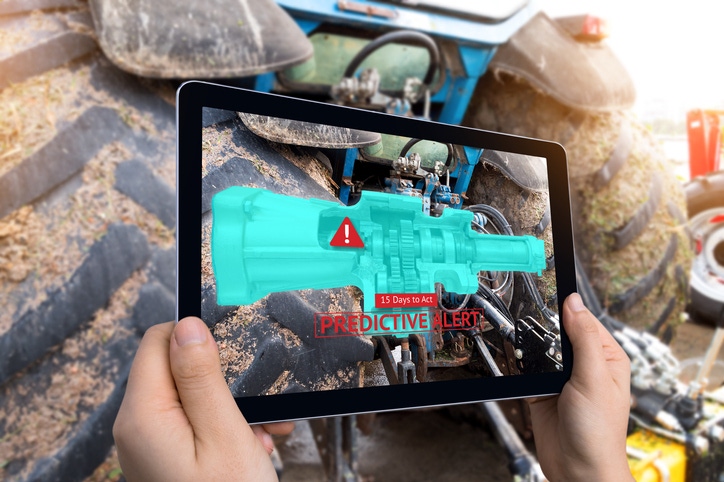
Wherever there is a discussion around the Internet of Things, the concept of failure is often close at hand. For instance, there is the threat of IoT-based cyberattacks to cause disruption in the physical world. Reports of hacked Jeeps to power grids to breached Iranian centrifuges have captivated the imagination. Conversely, one of the initial promises of IoT in enterprise and industrial contexts was to help predict the failure of a machine or business process. On top of that are reports that many IoT initiatives in recent years are failing. A 2017 Cisco study declared nearly three-quarters of Internet of Things projects had less-than-stellar results. In Cisco’s words, they were “failing.” A year later, McKinsey helped popularize the concept that many IoT projects were stuck in “pilot purgatory.” They might not be an outright failure, but they aren’t successful either.
This year, the IoT startup Particle performed a study to gauge the current state of the market. After surveying more than 800 professionals, many of these broader themes remain largely unchanged. “I wouldn’t say that the failure rate has changed in any meaningful way,” said Dan Jamieson, general manager of Particle’s enterprise IoT platform. Jamieson, however, points out that his standards for success are high. In his mind, a delayed IoT project, or an unexpectedly expensive one, is potentially a failed project.
Yet the Particle survey also revealed several sectors where respondents expected significant business benefits from IoT deployments. The majority of professionals in the technology, manufacturing, health care, energy and agriculture sectors expected their IoT products to lead to at least $1 million in financial impact.
Dan Jamieson
The top IoT use cases included remote monitoring (a focus of 78.4% of respondents), preventative maintenance (a priority of 54.7% respondents) and asset tracking (a focus of one-third of the survey takers).
Jamieson said there has been a gradual realization that the impressive-sounding concept of predictive maintenance can be difficult to execute. The concept seemed poised to be a star IoT use case, as Bain has noted. But predicting when a machine is going to break down is frequently difficult to do with a high level of accuracy. Industry professionals “were in this world where they told themselves: ‘We’re going to connect our machines and then we’re going to write a bunch of software that will analyze the machines. Before they break, a machine will tell us it’s about the break and we’ll send out a service person so there’s zero downtime,’” Jamieson said “That is a wonderful vision. And one of those things that we’re a long way away from.”
For context, a poll in the recent Fluke webinar “How Industry 4.0 and IIoT Drive a New World of Maintenance” asked the audience which type of maintenance the industrial audience focused on. A total of 54% selected “preventative” while 17% chose “predictive” maintenance. Another 23% selected single-variable condition-based diagnostics, which features the data measurement of predictive maintenance, but omits the algorithms that predict failure.
It wasn’t long ago that predictive maintenance seemed like a no-brainer. But Bain’s own survey of 600 executives found waning enthusiasm for the concept in 2018 compared with 2016.
The Bain report concluded: “Predictive maintenance is just one of many IoT use cases that customers have had difficulty integrating into their existing operational technology and IT systems. As companies in the industrial sector have invested in more proofs of concept, many have found IoT implementation more challenging than they anticipated.”
Particle’s research underscores that finding while echoing Bain’s conclusion that the long-term optimism around IoT projects continues to be high.
For now though, the paradigm for IoT-enabled maintenance is shifting for many organizations from predicting problems before they arise to simply detecting a problem quickly after it arises, according to Jamieson. What that means for manufacturers is they should be able to know when a machine is broken before their customers do. A company might be able to deduce a machine has broken down because of a change in current draw, for instance. And then they can dispatch a service person with the correct replacement part and repair equipment to avoid needing multiple truck rolls to address the problem.
That doesn’t mean the idea of predictive maintenance is outlandish. Over time, companies adept at preventative maintenance and field service management can lay the groundwork for accurate predictions. That is, they can amass the necessary data, analytics and algorithms to enable them to leverage machine learning or neural networks to declare when a machine is likely to break down. “They can say: ‘When the current pattern looks like this, we know that the machine is going to break down in six weeks on average with a standard deviation of seven days. So we’re going to dispatch a service person within that six weeks to replace that part so there isn’t any downtime from an end user’s perspective,’” Jamieson said.
The conversation around IoT security bears a certain amount of resemblance to that of predictive maintenance. In both cases, vendors have helped establish a narrative that preventing failure — whether in the case of a broken or hacked system — is straightforward with the right widget.
“But you can’t buy a product that is going to secure your IoT devices,” Jamieson said. “That’s nonsensical.” Security has to be woven into a company’s infrastructure and technology. It must pervade its culture and processes. “It is part of the fabric of what you do,” he added.
Enterprise companies’ business fabric should be rooted in common-sense thinking rather than buzzword-driven magical thinking. “We are forgetting business strategy 101,” Jamieson said. “Many people seem to be saying: ‘Don’t worry about the connectivity. Get the data, do AI and then profit,’” he said. “They spend no time asking: How do I get the data? What data do I want? How do I put the right infrastructure in place such that I can reliably create visibility into the machine in question?” Jamieson said. “They look at that like an afterthought, like: ‘Oh, cool, I’ll buy a chip. I’ll hook it up to this, and I’ll be good.’”
If businesses want to have success with the digitization of their business, they need to take a step back and ask questions that seem obvious but can be easy to overlook, Jamieson said. “Questions like: What problem are we solving? What don’t I know? What team do I need to build? How should that team be structured? What capabilities do I not have that I need to buy?” Jamieson said. If enterprise businesses came up with solid answers to questions like those, “I think they would have more success,” he surmised.
About the Author
You May Also Like