NXP Doubles Down on Machine Learning at the Edge
From Arm to Google to HPE to Microsoft, many tech heavyweights have worked to streamline machine learning at the edge. NXP is one of the latest to do so.
November 6, 2018
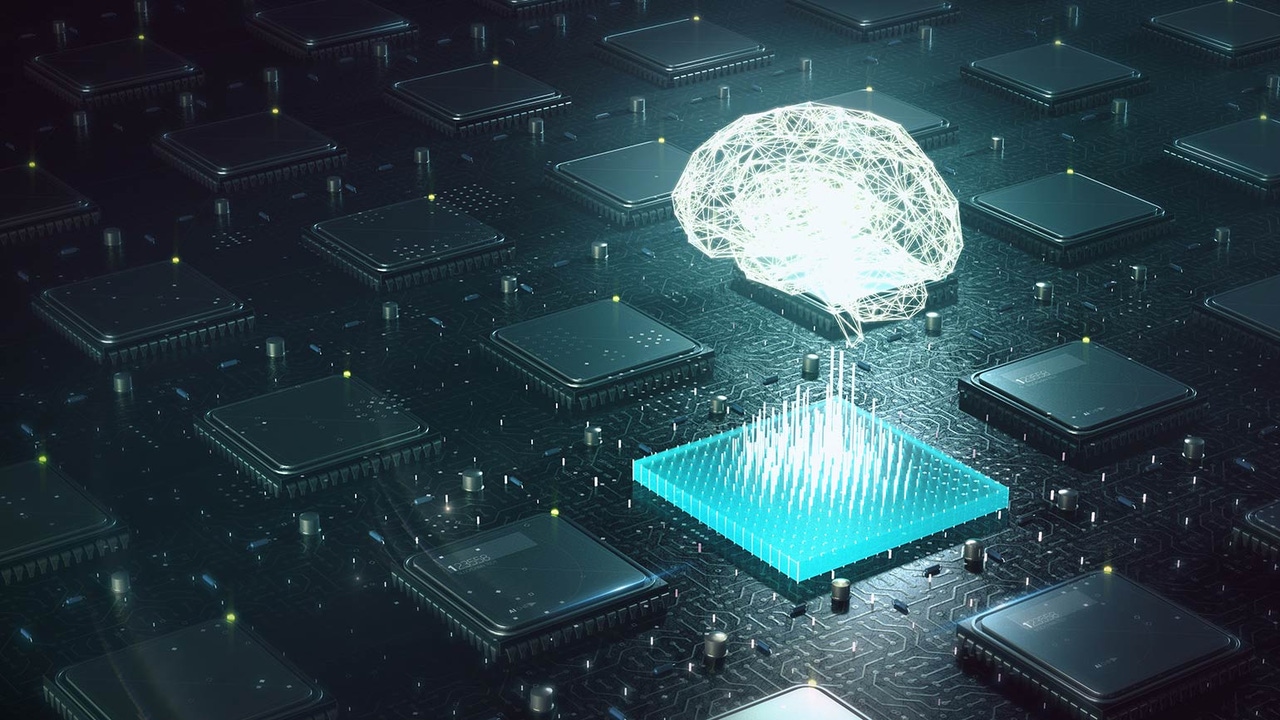
There are several things internet pioneer Robert Metcalfe is known for: Co-inventing Ethernet in 1970, co-founding the now electronics manufacturer 3Com in 1979 and for devising a widely-cited model to express the value of a telecommunications network. Known as Metcalfe’s law, the principle holds that the value of a telecommunications network can be calculated as the square of the number of networked devices. Despite criticism that the principle helped drive the dot-com bubble in the late 1990s, the principle continues to be used to describe the value of everything from the Internet of Things to social media networks and cryptocurrencies. In 2006, Metcalfe himself acknowledged that the principle hadn’t been “evaluated numerically,” unlike Moore’s law, which had decades’ worth of broadly supporting data. “Metcalfe’s Law is a vision thing,” he wrote. “It is applicable mostly to smaller networks approaching ‘critical mass.’ And it is undone numerically by the difficulty in quantifying concepts like ‘connected’ and ‘value.’”
Metcalfe also acknowledged it is possible that the value of a network could go down after it reaches a certain threshold. “Who hasn’t received way too much email or way too many hits from a Google search?” he has asked. “There may be diseconomies of network scale that eventually drive values down with increasing size.”
As the Internet of Things market grows, Metcalfe’s commentary points to the need to unlock value through optimal data sharing while avoiding drowning in “digital exhaust.”
[IoT World is the event that takes IIoT from inspiration to implementation, supercharging business and operations. Get your ticket now.]
“We are coming up against this obstacle where the more connected devices there are, the more unfiltered data that is coming into the network,” said Geoff Lees, senior vice president and general manager of NXP’s microcontrollers division. “We came across this fundamental idea that the power — the economic value of the network is proportional to the square of the number of devices that are on the network — Metcalfe’s law,” Lees continued. But unless all devices in a network are capable of securely sharing data, “we really don’t get the full value of the network.” And while the cloud is often a convenient location for data processing, it isn’t always feasible or possible to beam IoT to a remote location. “We’re discovering that there are many more applications in industrial and automotive that really were never meant to upload data to the cloud anyway,” said NXP Head of AI Markus Levy.
NXP’s solution to the problem, which it calls edge intelligence environment (eIQ), is a machine learning toolkit that can accommodate sensor stimuli from IoT networks. eIQ offers support for TensorFlow Lite and Caffe2 as well as other neural network frameworks and machine learning algorithms. eIQ takes the concept of machine learning at the edge and applies it to use cases targeting voice, vision, anomaly detection and so forth. “By installing an inference model at the edge, we’re essentially kind of aggregating the network’s knowledge and the network’s acquired data value,” Lees said. To support that goal, NXP vows to progressively increase processing performance at the edge with each successive generation of semiconductor technology, while also helping accommodate growing customer demand for security, data processing and local storage. “In the past couple of years, we have really evolved from the connected story to the how to increase edge processing capability,” Lees said. “We call it: ‘secure, aware and connected.’”
Examples of eIQ applications would be using computer vision to detect if industrial workers are wearing helmets, operating a piece of machinery incorrectly or are otherwise doing something undesirable. Especially for safety-related applications, the latency that results from sending data to the cloud and back is not tenable.
NXP’s plan to facilitate machine learning at the edge could help NXP’s customers unlock significant efficiency gains, Levy said. “I think it’s our job as a semiconductor vendor to provide this edge-computing/machine learning capability to our customers and make it easy for them to deploy.”
That goal also applies to cybersecurity. NXP is providing both hardware and software elements designed to make security more or less plug and play. “On the machine learning side, we’re doing the same thing,” Levy said. “We may be providing a cookbook, for example, that takes people through the steps of how do you deploy TensorFlow. [Our customers] are expecting us to solve this problem for them, and basically turn the whole machine learning concept into a form of middleware.”
Another barrier to machine learning adoption is cost, said Gowri Chindalore, lead strategist for embedded solutions at NXP. “A lot of our customers actually have trouble figuring out what is the system cost they have to incur in order to deliver a certain user experience,” Chindalore said. Some vendors may recommend higher end graphics processing units to support machine learning applications. Their high cost, however, can convince some implementers to conclude that machine learning is out of reach.
eIQ provides the ability for NXP customers to enter the specs they want to meet such as inference time to calculate what type of processor would be adequate for the application. “We are building that lowest cost option for your company to deliver what they need,” Chindalore said.
The company is also working with data analytics companies to develop not just modules that can be retrofitted into existing industrial environments. “An oil rig is absolutely a classic example for that,” Chindalore said. Mines are another example. “A lot of the mines need monitoring inside to detect poisonous gases and for miner safety,” he added. Such applications demand edge processing.
Cybersecurity is another consideration pushing processing to the edge, Lees said. “The larger the value of data that you hold centrally in the cloud, the greater the attack surface, the greater the attack value to all those malevolent hackers or organizations,” he explained. That is the reason NXP is making the case for distributed data stores with diverse access and authorization techniques and attributes. “As you carry that to the logical conclusion, you start to realize that the ultimate distribution is to maintain as much data as possible at the edge,” Lees said.
About the Author
You May Also Like