Trends Continue to Push Processing to the Edge for AI
Recent developments, including the NVIDIA-Arm deal and VMware’s Project Monterey, reveal big bets on edge computing.
October 13, 2020
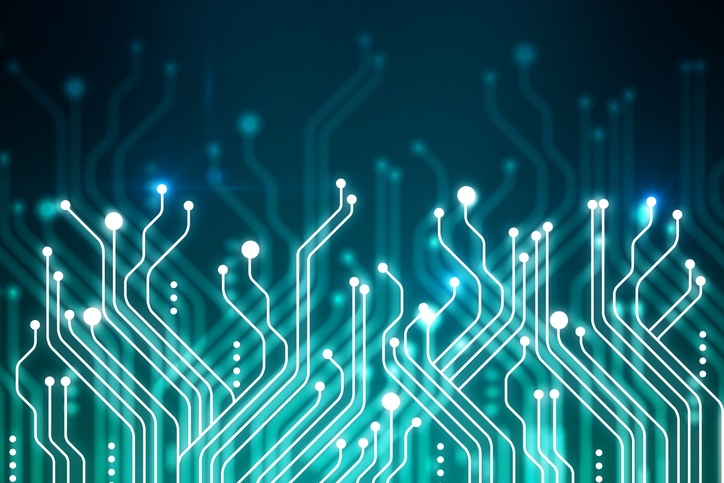
Key takeaways from this article include the following:
Device proliferation and data volumes now favor distributed computing models for speed, performance and data security reasons
Recent vendor moves by NVIDIA and VMware provide support for data-intensive processing, such as AI and machine learning, at the edge.
Many processes and industries will benefit from pushing processing to the edge, including Industrial IoT and consumer-based IoT.
As connected devices proliferate, new ways of processing have come to the fore to accommodate device and data explosion.
For years, organizations have moved toward centralized, off-site processing architecture in the cloud and away from on-premises data centers. Cloud computing enabled startups to innovate and expand their businesses without requiring huge capital outlays on data center infrastructure or ongoing costs for IT management. It enabled large organizations to scale quickly and stay agile by using on-demand resources.
But as enterprises move toward more remote models, video-intensive communications and other processes, they need an edge computing architecture to accommodate data-hogging tasks.
These data-intensive processes need to happen within fractions of a second: Think self-driving cars, video streaming or tracking shipping trucks in real time on their route. Sending data on a round trip to the cloud and back to the device takes too much time. It can also add cost and compromise data in transit.
“Customers realize they don’t want to pass a lot of processing up to the cloud, so they’re thinking the edge is the real target,” according to Markus Levy, head of AI technologies at NXP Semiconductors, in a piece on the rise of embedded AI.
In recent years, edge computing architecture has moved to the fore, to accommodate the proliferation of data and devices as well as the velocity at which this data is moving.
The edge computing market is expected to grow from $3.6 billion in 2020 to $15.7 billion by 2025, according to MarketsandMarkets data.
Market Moves Continue to Push Processing to the Edge: NVIDIA
Recent moves by NVIDIA, a giant in the graphical processing unit (GPU) market, and VMware, the virtualization vendor, support these new distributed processing architectures and clearly pave the way for lightning-fast processing at the edge.
First, in September, for example, NVIDIA announced its intention to pay $40 billion for Arm — in part to continue to push AI closer to the edge. While NVIDIA has dominated in the data center with its GPUs, Arm has a stronghold in the mobile market, supplying chips to Apple, Qualcomm and others.
“If you believe the future is AI, and you believe that is powered by GPUs and CPUs, then NVIDIA’s ability to create these end-to-end systems has just gone way up,” said Zeus Kerravala, in a video interview on the NVIDIA-Arm deal and prospects for AI at the edge. “Especially in the edge computing market — [NVIDIA] will be all over that.”
There are regulatory concerns about the NVIDIA-Arm deal, including whether it could imperil Arm’s successful licensing business. Arm has hundreds of licenses with its partners — which, in addition to competitors AMD and Intel — includes giants like Apple, Qualcomm and Broadcom. There are also serious antitrust concerns about NVIDIA’s newfound control of the mobile CPU market.
SmartNICs: Moving Processing Down to the Edge
Second, at the VMworld 2020 virtual conference in late September, VMware announced Project Monterey to provide improved AI performance with SmartNIC (or smart network interface card) technology and NVIDIA’s data processing units (DPUs). Project Monterey involves partnership with several companies, including NVIDIA, to bolster infrastructure to support AI applications at the edge.
Monterey offloads hypervisor, networking, security and storage tasks from a host CPU to NVIDIA’s BlueField DPU. Moving this processing to DPUs andSmartNics can forward AI, machine learning and other data-centric applications.
A SmartNIC runs non-application tasks from a server CPU, so the server can run more applications faster.
“There has been a general wave of interest in moving computation closer to the data,” said Alexander Harrowell, senior analyst at Omdia.
“It moves the abstraction of the hypervisor down from the host to the network … and offloads some of the work that the hypervisor itself does into that card,” Harrowell said. “It makes more of the processing power you paid for available. So there is a story here of a shift away from the classic Intel x86 model to the so-called Harvard Computing Model.”
This trend toward distributed computing, Harrowell noted, will continue apace and enable more complex processing, such as AI processing.
“By offloading a lot of that I/O work onto the Arm core and taking advantage of hardware offloads in those SmartNICs,” said Greg Lavender, senior vice president and chief technology officer at VMware,“you can offload that processing and free up even as much as 30% of the CPU … and give that back to the application so [it] gets the benefit of those extra compute and memory resources.
For the connected-things market, moving processing down the stack and into these distributed edge architectures will be a major boon for data-intensive processes that need rapid response times.
“Now that you can do AI at the edge, you can turn the IoT into something with real capabilities,” said NXP’s Levy.
>>>>>>>>>>>>>>>>>>>>>>>>>>>>>>>>>>>>>>>>>>>>>>>>>>>>>>>>>
For more coverage on Industrial IoT, take part in Industrial IoT World this December.
About the Author
You May Also Like