Startup Appnomic Aims to Enable Self-Healing IT Operations
The default for IT operations management is to wait for problems to arise before fixing them, according to Appnomic, which employs a self-healing approach.
November 12, 2019
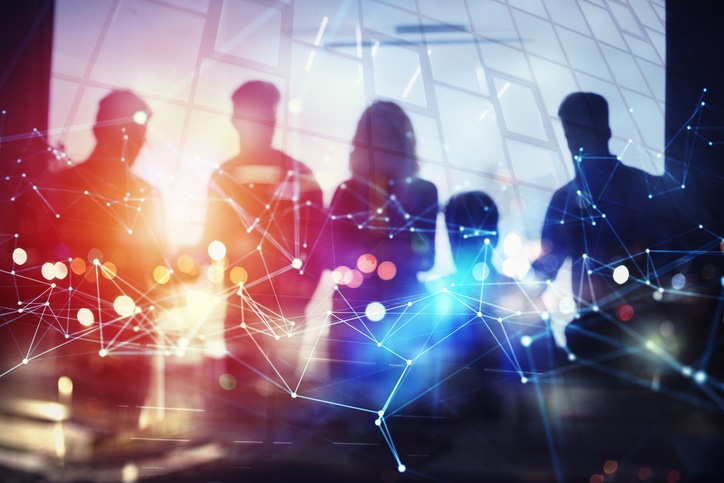
Heart disease remains the number one killer in the United States, responsible for one out of three deaths, but the way many people deal with the condition is to essentially wait until they have a heart attack or stroke before seeking treatment. Such a strategy is not only risky in terms of mortality risk, but it also comes at a cost of hundreds of billions of dollars each year.
While regular medical treatment is not a cure, it can help improve outcomes and reduce costs in the long- run. According to a 2001 report from Harvard economist David M. Cutler, “For every dollar spent on medical treatment of cardiovascular disease, the gain from people living longer is about $7.”
The way many organizations manage IT operations is broadly similar. In simple terms, the strategy is to wait until something bad happens, and then try to fix it. Advances in analytics and automation have enabled enterprises to accelerate the pace at which they detect and address problems, but the approach is still fundamentally reactionary.
The startup Appnomic, conversely, has a quest to enable what it terms “the self-healing enterprise.” The analogy of proactive heart disease treatment is a good fit for explaining the company’s approach, according to Cuneyt Buyukbezci, chief marketing officer at the company.
“Early detection is a big advantage,” he said. Just as a cardiologist who has diagnosed a patient with early heart disease would prescribe a treatment regimen designed to improve a patient’s long-term quality of life, Appnomic’s focus is similarly on preventing problems before they happen.
Launched in 2008 by the Bangalore-based entrepreneur Paddy Padmanabhan, Appnomic began with a fundamental observation. After witnessing a string of performance problems when implementing banking software, Padmanabhan wondered if the machine learning algorithms could trigger cognitive actions to prevent such complications from occurring in the first place.
Having worked for the past decade on refining its machine-learning algorithms, the company’s technology “works well with legacy as well as modern-day applications,” said Nitin Kumar, chief executive officer of Appnomic. It can ingest data from everything from applications, infrastructure, logs and so forth to create what Kumar referred to as “context awareness and intelligence from the technology landscape.” “We then later leverage AI and ML to create prediction and self-healing,” he added. ”Given we have a top-down approach i.e., workload-based, we can scale across environments.”
When asked what kind of timeline is necessary for the system to learn what types of IT operations behavior is normal and normal, Kumar said it can range from a few weeks to three months. It depends “on the nature and the complexity of the technology landscape,” he said. “The key thing here is to first build the baseline by ingesting data from multiple sources. The quality and completeness of that data is key to inform shifting baselines and anomalies.”
A Journey to Self-Healing
The pursuit of enabling autonomous IT operations is, of course, more ambitious, and involves an evolution, according to Buyukbezci. The first phase in that evolution is monitoring in the form of metrics, logs and alerts. Those items provide a baseline awareness of what is happening. The underlying question this stage seeks to answer is: What failed, and when did it fail? Understanding why an IT system failed, conversely, is trickier at this stage. “Once there is a failure, I can sift through thousands of lines of logs and try to figure out what might have caused that problem,” Buyukbezci said, explaining the modus operandi of this stage. It forces a user to look at data such as CPU use and hard disk capacity to develop a high-level understanding of IT operations while diagnosing problems to investigate.
Analytics are at the forefront of the second stage, which simplifies and streamlines data-gathering for IT teams. While primarily focused on past events, it also enables basic predictions and conclusions. This level is still “very much a rearview mirror,” Buyukbezci said,
Stage three involves AIOps (an abbreviation for artificial intelligence in IT operations) and automation. At this level, it becomes possible to orchestrate sets of rules rather than relying on a human operator. “If there is, for example, failure of a CPU, you can shift the load to another CPU,” Buyukbezci explained. Such automated functionality can help maintain business continuity. In terms of AI, enterprises can deploy techniques such as data clustering and correlation to streamline operations. As a result, an operator can now quickly sift through millions of lines of logs. Such tools can also help make deductions. “For example, the tool can correlate certain alert logs, group them and classify them as, say, CPU failure or an application performance degradation,” Buyukbezci added.
This stage of automation is a bit like a vehicle that combines adaptive cruise control with lane centering. While such vehicles can largely drive themselves in perfect freeway conditions, human input is still required.
In the IT realm, this automation stage involves using “using pockets of AI,” Buyukbezci said. It can streamline monitoring and failure management, but many IT operations continue to require human input. “There is still an operator looking at metrics and dashboards, but those metrics and dashboards have been made by AI algorithms,” Buyukbezci explained. While the process can streamline many operations, a human must deduce whether an alert the system triggers represents a real problem or a false alarm.
The problem of false alarms is significant. In the cybersecurity realm, for instance, 72% of chief information security officers cited alert and agent fatigue as a problem, according to 2018 Bitdefender research.
In Buyukbezci’s estimation, the bulk of IT vendors, including those offering AI and automation-themed solutions, are “pretty much waiting for something to break to fix it.” “Everybody measures the mean time between failures or the mean time to react to failures,” he added.
By contrast, Appnomic’s approach is predictive and preventive. The company’s marketing materials use the phrase “autonomous IT operations” to describe its goal. Instead of defining its offering in terms of response to a failure, Appnomic aims to eliminate IT problems in the first place.
Appnomic’s technology can trigger remedial actions specific for a given use case. “While all use cases can be automated, a few can be fully autonomous,” Kumar said. “As customers tend to get comfortable with AI assisting humans, they adopt the fully autonomous mode.” Kumar said there are some applications such as SAP where the company has code-level integration with the Advanced Business Application Programming programming language. In this case, it can create “autonomization across the development and operations,” Kumar said. “Our uniqueness is our ability to shape workloads, transactions, integrate with code (in specific applications), create context awareness and train the machine learning model to detect, predict and self-heal.”
Other organizations are also vowing to help enable autonomous IT. Hitachi Vantara and HPE, for instance, have indicated plans to help enable autonomous data centers. Google has deployed AI for autonomous data center cooling.
Autonomy “is the holy grail of running data centers,” said Martin Davis, managing partner of DUNELM Associates. Much like manufacturers are targeting lights-out factories and warehouses, “those running data centers are very keen to have self-healing infrastructure set-ups that can diagnose and resolve their own issues,” Davis said.
“The industry has been getting more and more automated over time,” he stated, while adding that most of the tools developed so far are designed to help operators rather than replace them.
In terms of organizations with IoT deployments, there are multiple angles where the concept of self-healing is pertinent, Buyukbezci said. One level is obviously ensuring such systems operate as intended, as well as any systems like a motor, pump, HVAC system and so forth, they may be connected to. Buyukbezci stops short of saying Appnomic can offer complete self-healing capabilities for such systems, but that it can be used to facilitate such an outcome. “The industry is using closed solutions. Every IoT vendor is coming up with their own monitoring,” he said.
Buyukbezci is not alone in that view. “IoT may be more difficult, due to the remoteness of the devices,” Davis said.
Another IoT-related focus for Appnomic includes projects deploying an edge architecture, software platform or back-end systems. “Then, all of the sudden, the IoT problem becomes an application problem,” Buyukbezci explained. “We can collect the data and process it and react to it.”
The company can help organizations with IoT projects bridge the IT-OT divide. That is, it can help unify the information technology back end with the connected devices deployed out in the field or on a shop floor. “From a self-healing perspective, we are able to collect the data from both operational technology and informational technology and unify them, if need be,” Buyukbezci said.
An Evolving Competitive Landscape
The concept of autonomous self-healing IT infrastructure is an attractive proposition. Time will tell whether self-healing infrastructure gains traction more quickly than, say, autonomous vehicles or the concept of preventing heart disease. “It is a little like everyone talking about autonomous cars for the past four to five years,” said Chris Kocher, cofounder of the consultancy Grey Heron. “Other than various limited tests in limited geographies, we have almost no completely autonomous cars in use today.”
The conceptual allure of self-healing IT infrastructure will likely mean more vendors will offer similar systems over time, Kocher said. Appnomic has won patent protection for several of its machine learning algorithms.
While in health care, paradigm shifts are relatively rare, as a result of factors such as ecosystem and stakeholder complexity, they are significantly more common in the IT sector. GIven the rapid pace of advances in artificial intelligence research in the past decade, the concept of self-healing IT infrastructure is likely to become part of conversations involving IT infrastructure management in the near future.
“There is a lot of ambition among IT vendors to deliver autonomous, self-healing networks,” said Daniel Newman, principal analyst at Futurum Research. “From Oracle’s autonomous database to Cisco’s Network Intuitive, we have entered an era of added productivity and efficiency that comes with deep automation and the application of AI and ML into common business workflows.”
Newman believes self-healing technology has a ways to go before it hits critical mass, “but the continued investment and innovation in this space coupled with a growing demand for automation will certainly be a catalyst for products like these to evolve and become the norm for managing and running enterprise IT,” he concluded.
About the Author
You May Also Like