GE Transportation and SAS team up on IoT edge computing for trains
Traditionally, analyzing data streaming from locomotives required considerable custom integration work. New IoT edge computing software from GE Transportation and SAS aims to simplify matters.
November 2, 2017
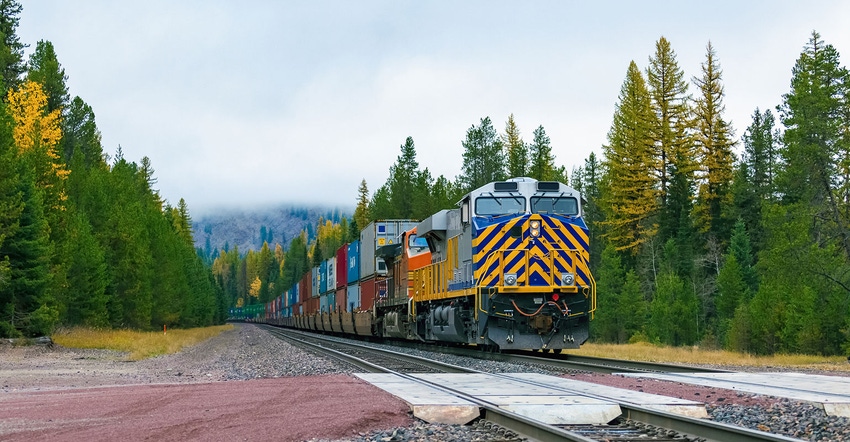
A modern locomotive can have up to 200 sensors collectively generating more than a billion data points per second. GE Transportation has been working to boost the IQ of trains with novel sensors and compute power in freight trains while also working to help train companies deal with the deluge of sensor data. In 2016, the company debuted GoLINC, a ruggedized IoT edge computing hardware platform designed to transform locomotives into mobile data centers. GoLINC weaves together an onboard computer with Intel processing power and communication modules while leveraging GE’s IIoT Predix software to sift through the sensor data to optimize train performance.
Last week, GE Transportation unveiled EdgeLINC software, which is device management software with an integrated Event Stream Processing analytics engine from SAS. The IoT edge computing software is designed to simplify local data analytics to bolster efficiency and reliability. “The challenge has been that there are often hard-coded rules running onboard or that the trains have to offboard a lot of data before it gets analyzed,” said Garret Fitzgerald, general manager of transport intelligence at GE Transportation. “The costs of offloading that data to do analytics in the cloud is either too costly, or it doesn’t work at all.”
By contrast, EdgeLINC with Event Stream Processing software from SAS is designed to enable train operators to use more data. “You can use a lot more of the data because you are doing the analytics right there,” Fitzgerald said. “You are not worried about offboarding it.”
One of the examples of how EdgeLINC can leverage data locally can be found in the temperature sensors on the train. If a baseline temperature on a train part is 90 degrees, it wouldn’t be helpful to have a stream of data stating that the temperature is fluctuating from, say, 89 to 91 degrees. “But when it spikes to 120 degrees, and you have created a baseline of 90 degrees, you can throw a flag,” Fitzgerald said. “You can run the analytics based on that streaming information coming off the asset.”
“Now that you’ve moved that assessment to the edge you can do some creative things,” said Jason Mann, vice president of IoT at SAS. Operators can, for instance, keep a running time window on the device. “Let’s say the window is a minute. When an event comes through that you want to trigger, you can just send a minute’s worth of data on each side of the event.” Contrast that with time-series data analytics, which looks at larger data volumes before it identifies anomalies.
“Running analytics at the edge is valuable, but to truly harness IoT data, analytics at the edge needs to be connected back to analytics in the data center,” Mann said. In particular, linking the edge with the data center supports learning throughout the product’s lifecycle.
[IoT Data & AI Summit demonstrates how the next generation of IoT and AI technologies will converge to unlock the intelligence of things. Get your ticket now.]
EdgeLINC also includes new tools for helping its customers manage edge devices and sensors on the train. “You might have 30 devices, and, with EdgeLINC, you can manage them as a single asset,” Fitzgerald said. “You can click ‘install’ once, and it applies an install template to all of those devices.”
The IoT edge computing software also makes use of Predix Machine, a software platform for embedded hardware linked to physical machines, and Predix EdgeManager, an edge computing platform that can accommodate data from up to 200,000 IoT devices.
While locomotives are its first application, EdgeLINC can be used for any use case with geographically distributed and mobile assets, including for freight shipping and trucking.
The system also supports autonomous engagement, so edge computing technology can not only remove the need to send data to the back office but it “can take the human totally out of the loop,” Mann explained.
It is likely that humans will still be involved for the time being, however. Most train companies want to see that an automated train technology is substantially better than a human before adopting it. “There is this very high burden of proof to go well beyond human capability,” Fitzgerald said.
For now, the North American locomotive market is focused on getting an ROI on the substantial investment in onboard computing in recent years to improve the reliability and efficiency of locomotives. Train companies are also entering into service agreements that are more focused on reliability rather than long-term maintenance agreements. “The industry has been spending billions putting compute onto their assets just to be compliant with regulation,” Fitzgerald said. “Now, they have a level of maturity that they have been forced into managing all of that compute and sensors.”
About the Author(s)
You May Also Like