Precision of Digital Twin Data Models Hold Key to SuccessPrecision of Digital Twin Data Models Hold Key to Success
As the industrial sector turns to digital twin technology for operational efficiency, digital twin data model accuracy is key to success of digital replicas.
January 4, 2021
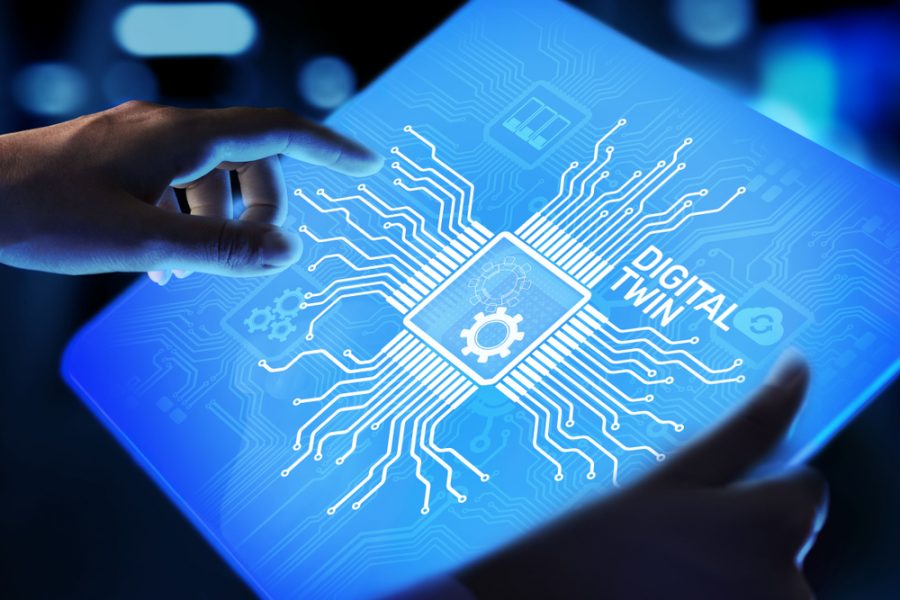
Key takeaways from this article are the following:
Digital twins are still nascent but accelerating digital transformation in the industrial sector and elsewhere.
COVID-19 has given digital twin technology new relevance, aiding in such tasks as remote monitoring, predictive maintenance and automated processing.
Reliability of digital twin models hinges on the precision of digital twin data models, however.
Digital twins are important tools as companies travel the road of digital transformation. Despite a science-fiction-like status, digital twins have started to emerge in real life, and in industrial and other settings.
While digital twins remain nascent, they have already begun to aid enterprises’ digitization efforts dramatically. Definitions remain fluid in the still-developing field. For some, a digital twin is a prototype in the design phase, an instance when it is deployed, or an aggregate when it is combined with other twins in operations. Some cut the cake differently, categorizing twins as asset twins, network twins or process twins.
Consider some of these uses of digital twins and their convergence with Internet of Things (IoT) devices:
Advances take diverse forms: The Rotterdam Port of Authority has used IBM digital twins and sensors to predict efficient mooring and departure times.
Power industry asset manager CPV uses GE Digital performance management software to create a digital twin of critical plant processes, while applying AI to up capacity and cut fuel use.
Ford has integrated predictive digital twin technology into its automotive powertrain manufacturing processes.
Atos and Siemens undertook a digital twin pilot that is part of big pharma efforts to respond to critical COVID-19 pandemic production pressures.
These and other digital twin examples combine several distinct technologies, including computer simulation, product lifecycle management, software modeling, virtual and augmented reality, robotics, machine learning and more. Digital twins create increasingly sophisticated virtual software representations of objects and systems, and feedback from their “digital threads” can inform design options. That data between products, processes or models in some cases can actively guide end-to-end operations.
Today, the digital twin in industrial IoT presents IT managers with a new take on familiar tradeoffs. There is opportunity, but there is also risk on this frontier – as implementors must carefully distill high-tech vistas into their own digital twin use cases.
Digital Twin Tech: Long-Brewing Overnight Success
Like most “overnight success” stories, digital twins are not new. NASA’s use of simulation in the Apollo space program carried the seeds of digital twin technology. The foundation for today’s twins was much enabled by advances in computer-aided drafting (CAD) and volumetric modeling that go back to the 1970s. The term “digital twin” began to gain currency 20 years ago in the area of product lifecycle management. Today, GE counts more than 2 million digital twins in production. Tesla is said to create a digital twin of every car it sells.
But if digital twins have a long-storied past, they have found new relevance in the present. COVID-19 has placed a new spotlight on digital twins. Remote asset monitoring, predictive maintenance, and process automation were already apt targets for twins, but COVID-19 has provided further rationales.
A recent Gartner survey Indicated that 27% of companies plan to use digital twins as autonomous equipment, robots or vehicles. The COVID-19 experience is a big driver. The research firm expects that by 2023 one-third of medium-to-large-sized companies with IoT deployed will have implemented at least one digital twin that is motivated by a COVID-19-related use case.
The Father of Digital Twins
What is new for today’s digital twins is that they can enlist the Industrial Internet of Things (IIoT). Exploiting IoT sensor data is a key to the next stage in digital twins, according Dr Michael Grieves. He is widely credited as the first to put forward the digital twin concept in manufacturing, laying the groundwork in 2002.
IoT was front and center when Grieves spoke recently at the American Society of Mechanical Engineers’ (ASME’s) Digital Twin Summit. There is work ahead, he advised.
“We need to basically be able to start collecting the IoT information from the products themselves, and pull that information together,” said Grieves, now chief scientist for advanced manufacturing at Florida Tech.
He said digital twin data gathering today is mostly ad hoc, but focus will soon center on a digital twin’s integration within a larger factory setting. The next step for digital twins, he said, is “operational sustainment.” Combining AI and machine learning with real-time sensor data will usher in “the intelligent digital twin,” in his estimation. With such implementations, a digital twin moves further away from its roots in simulation and prototype modeling, and deeper into ongoing operations.
Digital Twin Data
Potential for digital twins in industrial settings revolves mainly around creating operational efficiencies. Small savings in resources, process steps, or downtime can scale into large savings throughout an enterprise.
Successful implementation relies on careful consideration of fidelity; that is, the level of precision of parameters that a digital twin transfers between the physical and virtual domains.
Fidelity is closely related to the accuracy of digital twin data collection, which must be carefully managed based on use case. Not all data is necessary for a digital twin to do its job, but narrowing the focus of data collection may require trial and error.
And, as always, a clear-cut business objective must drive the overall effort, industry watchers have emphasized.
The question, according to Dan Isaacs, vice president and director of the CTO Digital Twin Consortium, is “What job is the digital twin being hired to perform?”
“We have seen a mix of approaches. But it is best to start with a clearly defined problem, to pursue objectives such as to increase productivity, and decrease downtime, to focus on what is feasible,” he said.
Issacs described the Digital Twin Consortium as a program launched by the Object Management Group (OMG) to achieve standard definitions and combine cross-industry efforts. He said the group has grown to nearly 150 members since its formation earlier this year. Its member list includes Autodesk, Bentley Systems, Dell, GE Digital, Microsoft, Northrop Grumman, and the University of Maryland.
With the digital twin, implementors and architects alike need to understand the latency requirements of systems, according to Said Tabet, chief architect in the CTO Office at Dell Technologies, and board member of the OMG’s Industrial Internet Consortium.
“Not everything is real time and not all real time is equal,” Tabet said. In other words, pursuing ultra-real-time response rates may not be feasible, or necessary.
Meanwhile, paying attention to the synchronization between the physical and the digital is also important.
“Implementing a maturity model will help guide this process. And, when it comes to [data] granularity, it’s important to focus on the outcomes and initial results as these will vary depending on use cases,” Tabet cautioned.
Supply chains and logistics are areas where digital twins are finding footing, and, in terms of modeling, they provide examples of how decisions on digital twins’ fidelity to physics can be set, said Sameer Kher, senior director for digital twins at Ansys, maker of Twin Builder software.
“In the supply chain, there are critical components, and some of these need modeling at a deeper level of fidelity,” Kher said. ”Products have physics.”
As an example, he pointed to hand sanitizers, the object of well-reported manufacturing line conversions during the first days of the COVID-19 pandemic.
Measures of the viscosity of fluids that comprise hand sanitizers — and the back pressures they can place on equipment — could be a vital part of digital twin production monitoring.
Digital twins in design stages allow what-if analysis that improves operations, he said.
Don’t Overthink Digital Twin Design
Potential users should be wary of overreaching when creating digital twin models, according to Jim Tung, fellow at MathWorks, maker of Simulink and other modeling tools.
“Sometimes, a full-fidelity, discrete simulation model of an entire workflow is just overkill,” he said. Users instead might focus on particularly expensive assets. Moving these elements more quickly through a production queue can reap cost benefits.
“In every case, it’s important to understand the business value,” Tung said. That is because users are led from that decision to answer questions about the proper fidelity of the digital twin model, he indicated.
As impressive as digital twin technology can be, technology is not the point, reminds Niels Thomsen, who heads the Insight Practice for IoT and AI at Atos SE.
“You have to start with the business angle,” he said. In Atos’ work in pharmaceutical industries that has meant starting with critical processes that determine the overall quality of chemical batches.
That requires building a digital twin model to measure against, comparing actual chemical mixes in real time with that model, and — in some cases — automatically adjusting parameters such as temperature, pressure and speed of flow to improve the resulting quality of mix.
Digital Twin Maturity Modeling
The course to today’s digital twins has been a long one for leading automotive manufacturer Ford, according to Dr. Annie Zeng. Ford started on this path 30 years ago, said Zeng, recalling the days of “C3P,” which stands for CAD/CAM/CAE/PIM, or computer-aided design/computer-aided manufacturing/computer-aided engineering/product information management.
Today Ford has models of both parts and processes in place, so that twin models of key car components can be monitored in the context of overall models of factory operations, said Zeng, technical expert for digital twin and AI at Ford’s Advanced Manufacturing group. Zeng, like Grieves, spoke at the ASME Digital Twin Summit.
For Ford, Zeng said, a digital twin is really a collection of data representing the product and production.
“Our vision is to provide timely access to — and insights — from relevant data,” she said. That means working with teams to uncover which data points are most timely and relevant to their quest for efficiencies. The project begins with asking teams to sit down and discuss what data is most immediate and useful.
In Zeng’s experience, digital twin builders must gauge the relative maturity of the technology being considered. According to Zeng, questions to ask are “Is it now? Is it near? Is it far?”
“The answer will be different depending on what your company wants to achieve,” she said. Other questions to address are whether you want to develop the full technology internally, or, alternatively, which part of the technology you want to develop in-house.
The answers to these questions should account for what type of development or deployment framework a user has in place. “I bet every company will have some sort of framework,” Zeng remarked. “If you don’t, you better have one.”
Digital Twins Aren’t Video Games
As part of the ASME summit, digital twin visionary Grieves was asked what business leaders should know before taking on digital twins.
“First of all, they need to understand it’s not a video game,” Grieves said. “It actually is about data coming in from real life and being able to do something with that.”
Data collection must be approached with common sense, he maintained. So, it is not a question of collecting every data point.
“One of the things that I warn against is [collecting] massive amounts of ‘data’ but no ‘information,’” he said. Thus, it is key to look at what kind of data is specifically needed in each instance.
Most important in conversations with the business side, said Grieves, is to focus on use cases where trouble points are already common knowledge.
“Where do you get value? If the businesspeople think, ‘I have to spend all this money to just get a pretty picture of something,’ they’re not going to do it,” he said. “You’ve got to basically bring this down to what the value proposition is.”
Still, “You can’t let the accountants get in too early,” Grieves adds with perhaps a bit of a wink. “They are the killers of joy.”
About the Author
You May Also Like