The Power and Pitfalls of Digital Twins in ManufacturingThe Power and Pitfalls of Digital Twins in Manufacturing
Digital twins will be vital for industrial organizations in the future, but deploying them successfully won’t be easy.
February 20, 2019
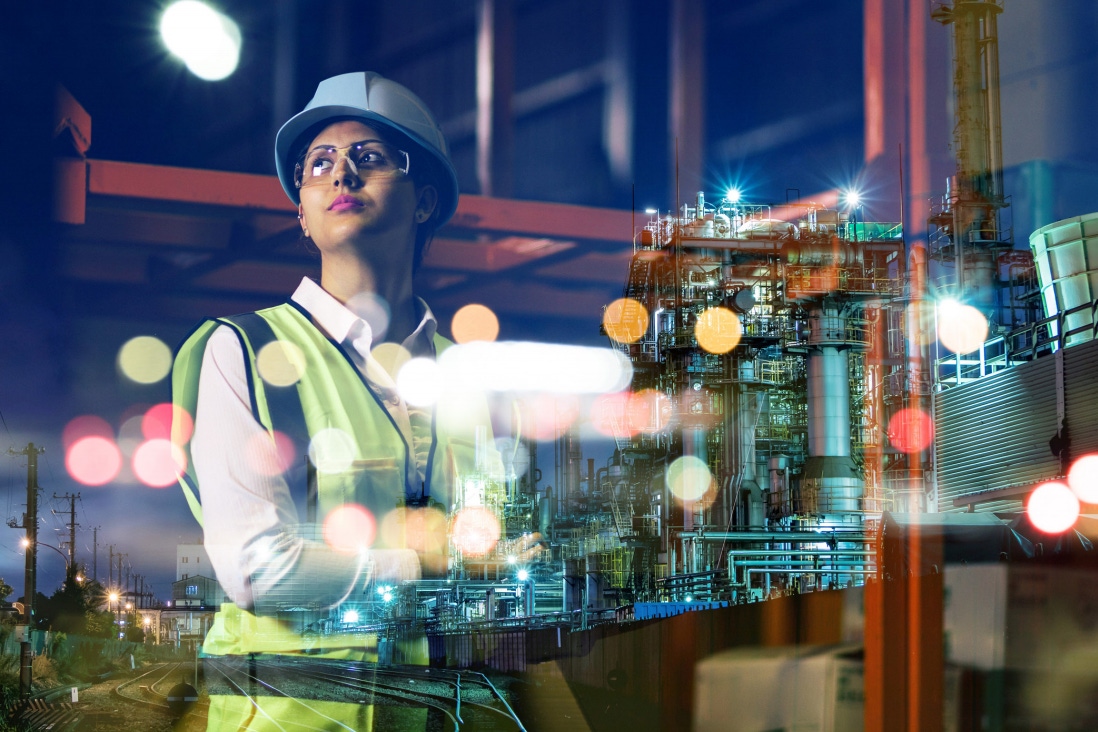
A decade from now, there will likely be a digital twin for consumer devices, industrial machines, the electrical grid, oil and gas infrastructure and even entire factories. But while digital twins are hot now, it is not always clear precisely what is meant by the term. While it is clear that they are data-based representations of physical objects, the resolution of current digital twin projects varies considerably. “There are digital twins, digital almost-twins, digital ‘look-alikes’ and digital ‘wish-we-were-related’-type degrees of twinness,” said Dan Miklovic, a research fellow at LNS Research. “We see lots of companies using very small ‘DNA strands’ of digital twinness today with the technology becoming far more important over the next few years.”
In early 2018, the digital twin is still an emerging technology, although its roots stretch back to 2003, when Michael Grieves, Ph.D. introduced the concept in an executive course on Product Lifecycle Management at the University of Michigan. Last year, Gartner pegged the technology halfway up the initial ascent of the “Innovation Trigger” stage of its hype cycle, which looks rather like a roller-coaster lift hill whose peak is dubbed the “Peak of Inflated Expectations
Because digital twins are a nascent technology, many of their promises remain fuzzy and intertwined with the marketing pitch for technologies such as IIoT, analytics, machine learning, artificial intelligence and cognitive computing. The basic idea is that the digital twin can bolster productivity and optimize physical assets, processes or systems. “The digital twin is the best way today for industrial companies to simulate and experiment without touching a production system. You can create models using precise real-time data. This is something extremely new,” said Poniewierski.
In addition, digital twins can simultaneously spur collaboration among workers who, after using digital twins to spot a problem, can pool their resources to address it. Proposed benefits of digital twins in the industrial space include the following: helping manufacturers improve the quality of finished goods, bringing predictive maintenance capabilities to manufacturing equipment, assisting manufacturers in the transition from selling industrial outcomes rather than discrete products while helping industrial organizations optimize their machinery, products, production lines or entire facilities. It so happens that all of those benefits have been directly linked to IIoT itself.
One of the chief benefits of digital twin technology is its potential to drive experimentation. “A manufacturer could build a digital twin for, say, a predictive maintenance application. They can then train the real behavior of the machine for their operators, machines and suppliers, in essence building a real-time test bed for improving the quality of production,” Poniewierski said. “They might realize that experimentation on live digital twins will cost, for example, one dollar, whereas running that same experiment in a development environment will cost $100.”
According to Gartner, a digital twin has four essential features, all of which are closely related to the “thing” in an Internet of Things project. First, there is a model of the “thing” it represents. Second, there is data about that thing such as its identity, status and context. Third, there is a twin’s uniqueness. In other words, each digital twin corresponds to a unique object. Finally, the digital twin monitors the status of an IoT “thing.” “You can ask the twin for information about the thing,” explained Nick Jones, vice president, distinguished analyst at Gartner at the November 2017 Gartner Symposium in Barcelona. Digital twins can also optionally be equipped with analytics for applications like predictive maintenance. There is also the option for digital twins to control physical objects. Lastly, some digital twins are capable of simulating the “thing” they are meant to replicate.
To be clear, the idea of using sophisticated models of physical products is not new. Organizations such as NASA have been using advanced simulations for spacecraft for many years. What sets digital twins apart is their connection to connected sensor data streaming from IoT-enabled objects, enabling them to discover insights and trigger actions based on sensors and smart machines and, in factories, manufacturing execution systems, eroding the barriers between the physical and the digital worlds. While the concept of bringing those physical and virtual models together is not new, it has been incomplete, as Grieves observed.
Manufacturers can also deploy digital twins that put AI/machine learning capabilities at the edge of a network to detect anomalies and spot data patterns next to a sensitive piece of equipment on the shop floor.
Specific use cases for digital twin technology have also been proposed and in some instances realized. For instance, digital twins have the potential to help industrial companies reduce the expense of product development and remote maintenance tasks. In addition, companies relying heavily on destructive testing during product development could perform a significant portion of such virtually. For instance, an automotive company could use digital twin technology to reduce the need for crash testing prototype vehicles. Similarly, companies relying heavily on building elaborate models and prototypes could save money by using digital twins during product development. Furthermore, organizations operating valuable assets in remote locations could use a digital twin of the equipment to simulate service life, helping curb the need to send a technician to that site to check its status. Digital twin technologies also could help manufacturers improve the level of sophistication and usability of future project generations while also helping speed time to market.
Digital twins are also at the heart of a whole suite of proposed applications in which technicians donning AR visors can gain a virtual view of what is happening inside a piece of production equipment. Digital twin technology could also help guide an inexperienced technician equipped with an AR headset to spot defects and learn to perform routine repairs thanks to step-by-step instructions that break the tasks into discrete steps that show, for instance, which screws or bolts to remove, the proper orientation of a new component and so forth.
While an array of benefits have been proposed for the technology, one of the impressive aspects of digital twin technology is its potential to scale. A first-generation digital twin could, for instance, help the owner of a wind farm optimize an individual wind turbine. A second-generation turbine could help optimize the entire wind farm, while subsequent generations would, thanks to increasing resolution, enable further gains while also allowing the turbine manufacturer to refine its latest product line using production data.
While it may seem that mainstream digital twin adoption is at least several years out, many organizations are already deploying them. While Gartner estimated last year that mainstream digital twin adoption was another five to 10 years out, 48 percent of enterprise companies implementing IoT technologies are “already using or planning to use digital twins in the next 12 months,” Jones explained.
In 2018, LNS Research expects digital twin initiatives to focus on replicating industrial processes rather than just the physics-based aspects.
Digital twins could also be a boon to artificial intelligence research. One of the reasons AI research stalled in decades past, according to AI pioneer Marvin Minsky, is because of computers’ limited sensory input and ability to interact with the physical world. Because digital twins align machine learning and AI with sensor data, that barrier could be steadily overcome in the next several years as digital twins become mainstream. Digital twins can help create a sort of digital feedback loop between connected products and machines and their environment and user base.
Ultimately, digital twins promise to be a powerful technology that, when used in conjunction with IIoT, can help transform the industrial landscape. Yet both IIoT and especially digital twin technologies remain at an early phase of adoption, and, for many organizations will require considerable customization work to create bespoke digital twins for their unique operational needs. That, in turn, will likely require the expertise of experts in fields like machine learning and artificial intelligence, who command salaries in the six- and even seven-figure range. Even recently graduated machine learning doctorates can command starting salaries in the $300,000 range. On the flipside, a growing number of vendors are beginning to launch digital-twin related services or products, which could contain costs of the technology to some extent, and their potential to curb physical testing while driving efficiency gains could quickly offset the initial capital outlay needed to deploy the technology.
High-resolution digital twins could also become a valuable asset to whoever possesses them — whether it is the industrial company or a cybercriminal who gains access to the technology. “Now, with IIoT and digital twins, you integrate both knowledge and experience,” Poniewierski said. “It is a killer mix.”
About the Author
You May Also Like