Volkswagen Explores Quantum for Machine Vision, Scheduling
A Q&A with Terra Quantum’s Florian Neukart, the former director of Volkswagen’s Data Lab
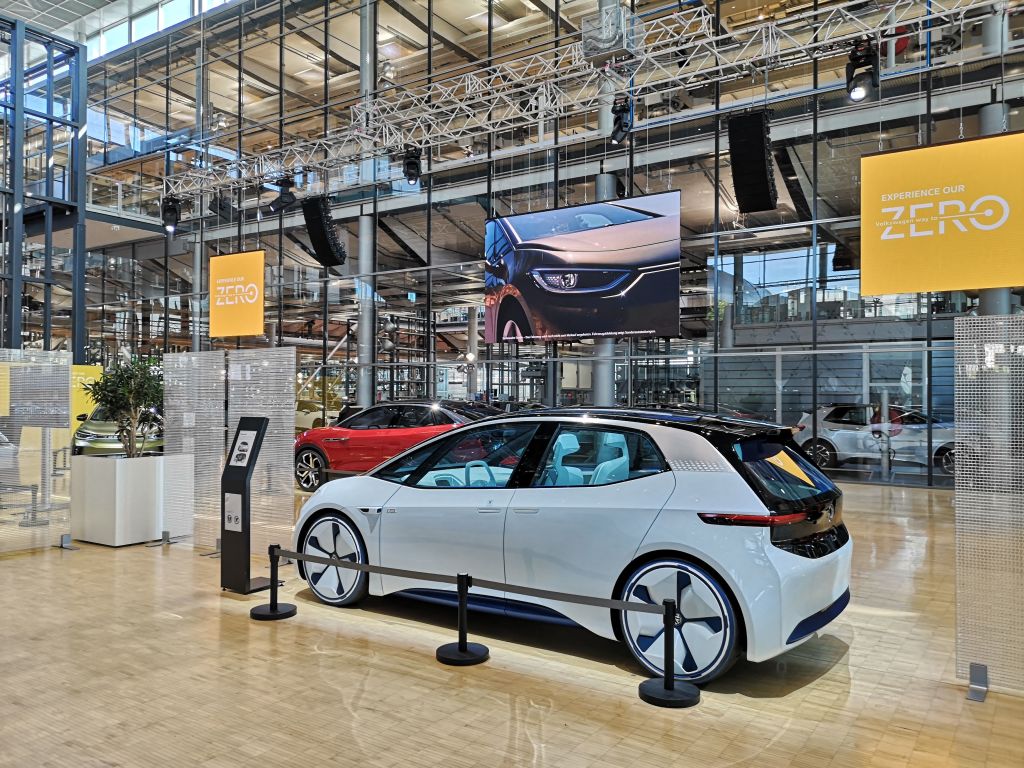
Carmakers have many uses for image recognition, ranging from assembly plants to training grounds for autonomous vehicles. Researchers at Volkswagen, Terra Quantum and Leiden University have combined classical and quantum computing to make the training process for these algorithms faster and more efficient.
The research focuses on two specific tasks: a quantum-inspired hyperparameter optimization technique and a hybrid quantum-classical machine learning model for supervised learning. The researchers published their paper in Quantum Machine Intelligence.
Florian Neukart is the chief product officer for Terra Quantum and the former director of Volkswagen’s Data Lab. In this exclusive Q&A with Enter Quantum, he discusses the research, Volkswagen’s early involvement with quantum computing and other applications for quantum the carmaker is investigating.
Florian Neukart is the chief product officer for Terra Quantum and the former director of Volkswagen’s Data Lab. In this exclusive Q&A with Enter Quantum, he discusses the research, Volkswagen’s early involvement with quantum computing and other applications for quantum the carmaker is investigating.
Enter Quantum: How did Terra Quantum come to work with Volkswagen on using quantum computing for machine vision applications?
Florian Neukart: We decided to provide Volkswagen with algorithms that can be used for multiple use cases but wanted to start with a concrete example. We selected image classification, which is a very prominent task for Volkswagen in many different areas, starting from production, where you have automated detection of defects for example, or image classification when it comes to self-driving vehicles and environment perception.
We had a data set that was the different vehicles that we wanted to identify with quantum-hybrid architecture – using quantum computer plus classical computers to train the neural network – and compare that with the same architecture that has only been trained with a classical computer.
We found significant advantages, achieving both faster learning using fewer instances to get equally good results, and the result was better than the purely classical approach.
You have a background working in Volkswagen’s data lab. How did that influence this project?
When I was at Volkswagen, we started a quantum computing group in 2015. One of the first things we did was traffic optimization, taking real-world data and processing it using a quantum annealing system from D-Wave. It didn't solve all the traffic problems in the world, but we showed that you could solve a real-world problem back then.
That matured into many other things and image classification was one of these, but this particular approach that Terra Quantum took with Volkswagen is entirely new.
The research involves hyperparameter optimization and a supervised learning model. How do these work together to help improve the machine vision process?
The hyperparameter optimization is conducted using a quantum neural network. A quantum neural network does not have a fixed structure, so it can have a variational layer or many variational layers. This means that you optimize the parameters of this layer with a classical algorithm, but then you execute it on a quantum computer.
The output data produced by that quantum neural network serves as an input to the residual classical neural network. So the output is used to optimize the hyperparameters of that classical resonant neural network.
Effectively, you feed the data into the quantum neural network, take that output, feed it into the classical resonant architecture, and so it is perfectly configured to handle the data directly. When we execute, then the quantum neural network is not used anymore, it's only used for training.
What other use cases is Volkswagen using quantum computing for?
They have many machine learning problems that go beyond image classification, such as prediction of market behavior. We also worked with Volkswagen on a scheduling optimization problem.
Car manufacturers test vehicles when they come off the production line. Some of the tests result from field errors or customer complaints. You have to test a specific model because customers tell the workshops that every time it rains, I get a wet seat, for example. So Volkswagen would have to test for water damage.
But there are I think about 250 test classes with many sub tests that you can do, likewise for braking and any other thing that you could test in a vehicle. Ideally, if you had enough people and enough time, you would test everything, but you can't, so you randomly select a few tests.
You do not have an unlimited number of people in production and all these people have different skills. So if I have two people, it's very unlikely that they are capable of doing the same tests based on the skills that they have. And it even differs from day to day; not everyone who is here on Monday is also in on Tuesday. How do I now take people and their skills and maximize the number of tests that I can do per vehicle? That’s the goal.
It doesn’t sound exciting, but mathematically this kind of scheduling problem is impossible to solve for classical computers. That's why we used a hybrid quantum approach and achieved a very good result.
This article first appeared in IoT World Today's sister publication Enter Quantum.
About the Author
You May Also Like