Tapping AI for Intrusion Detection SystemsTapping AI for Intrusion Detection Systems
AI has enabled intrusion detection systems to be adapted for IoT networks, which have been difficult to cover with traditional alternatives.
October 18, 2021
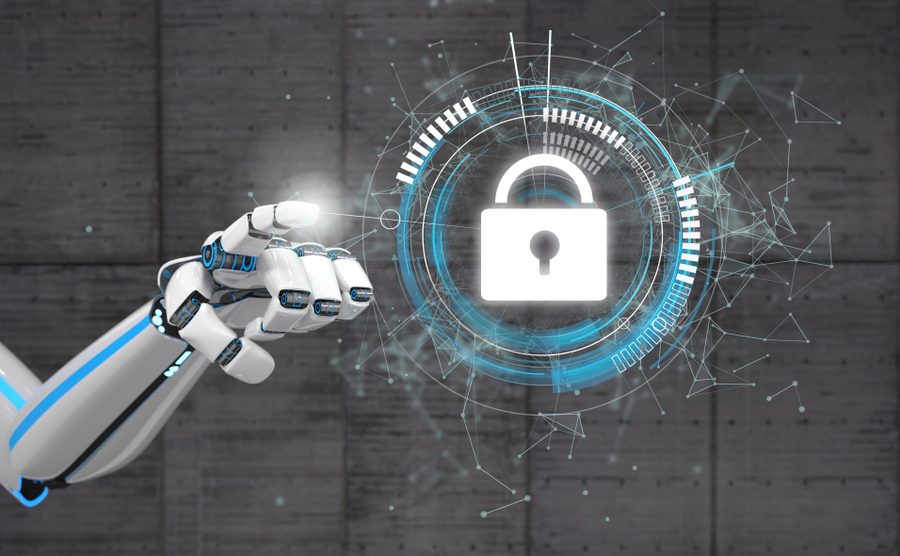
Internet of Things (IoT) devices have increased the attack surface, creating additional entry and exit points for any systems within which they are used.
Typically, though, traditional intrusion detection systems (IDS) are primarily rule-based and they’ve not been able to keep up with emerging threats, which are constantly launched through, from and against IoT devices, said Rebecca Herold, IEEE member, CEO and founder of The Privacy Professor consultancy.
“As it relates to IoT, an IDS needs to not only monitor the IoT device itself but also identify threats from the other components involved with the full IoT product,” she said.
So, the IDS would need to treat the following as potential intrusion sources: IoT back-end systems, such as the supporting cloud services, along with the mobile applications interfacing with the IoT device, the local hub and possibly other remote hubs within the IoT device ecosystem and any other type of connecting element, Herold said.
Rule-based IDS vs AI-based IDS to Detect IoT Attacks
Rule-based IDS will look for known attack behaviors and alert on them (like standard signature-based IDS) while artificial intelligence (AI)-based IDS will look for deviations from a behavioral model acquired by the AI algorithms, said Ariel Zeitlin, co-founder and CTO, Guardicore.
However, rule-based approaches will always fail in the IoT as we have very few attacks to examine, according to Jamison Utter, senior director product and solution evangelism for Ordr.
“The field is so new that so far, we see password attacks and a few specialized industrial attacks,” he said. “All IoT threats will be and should be considered ‘unknown’ and ML [machine learning] is the way to detect that. The good news is devices are deterministic, in that they act and perform the same tasks, the same way every day—otherwise, they are broken. Therefore, it’s a fantastic application for AI/ML technologies.”
How Do AI-Based IDS Work to Detect IoT Attacks?
AI-based IDS are in their infancy and, in general, are certainly still an emerging type of product for securing IoT products, Herold said.
“What makes AI-based IDS an attractive option is that AI can be more nimble and effective within the wide range of ecosystems where IoT devices are used,” she said. “For example, within extremely scalable cyber-physical systems where there are many IoT devices that may be connecting and disconnecting to the ecosystem throughout any point in time and where continuous data analytics are being performed throughout a complex wide area network.”
Rule-based IDS look for specific signatures or set patterns in the traffic, for example a particular command, key words and/or traffic patterns, said Scott Laliberte, managing director, emerging technology group, Protivit.
An AI/ML-based IDS attempts to learn/benchmark the normal or typical forms of network traffic generated by IoT devices and identify anomalies based on algorithms and deviations from those normal or typical forms of traffic, he said.
Is a particular device acting differently than it normally does or different from its peer group? Does a combination of actions or attacks look similar to other attacks or indicate a possible attack may be developing?
“An AI/ML-based IDS can be very effective, if developed properly, but it requires solid algorithms, good data sets for training and expertise to adjust and improve the model,” Laliberte said. “AI/ML-based methods require tuning by operators to make them valuable.”
AI-based IDS will usually try to establish a model of normal behavior of connected devices and then detect deviations from this behavior, Zeitlin said. In particular, it may try to identify the devices by fingerprinting them from the network and then identifying deviations of the typical behaviors of such devices in the real world.
Benefits of Applying AI-Based IDS to Detect IoT Attacks
AI-based IDS systems are superior in their ability to identify threats autonomously, which is typically done with machine learning models. Their accuracy rate can range from the 80 percentile up into the low 90 percentile, said Chuck Everette, Deep Instinct’s director of cybersecurity advocacy.
“Deep learning, an advanced subset of machine learning, can get the accuracy rating up to 99% with the proper prevention solution,” he said. “With the proper training, deep learning can think like the human mind and make decisions within milliseconds, deciding if a file or network flow is malicious or benign.”
Using soundly engineered and thoroughly tested AI-based IDS can help identify signs of possible intrusions through, or attacks being launched from, compromised IoT devices sooner than previous generations of IDS, according to Herold. This can then help stop widespread access through the digital ecosystems where compromised IoT devices are located.
“AI-based IDS can also help enable defenders to take action more quickly to slow down attackers,” she said. “Well-engineered AI-based tools can automate the detection of attacks at the edges of a network as well as those launched from inside digital ecosystems.”
Challenges Applying AI-based IDS to Detect IoT Attacks?
The challenges in developing and deploying an AI/ML-based IoT IDS are how early we are in the IoT maturity cycle and the inconsistent implementation architectures that can make effective use of AI-based IDS difficult, Laliberte said.
“A lack in adherence of protocols and standards in IoT makes it more difficult to develop effective AI and gather sufficient data sets to train the models (your data sets would have to have sufficient data with the different protocols, device types, architectures, etc.),” he said.
In addition, the demand for IoT IDS is still developing. Many organizations do not even have general governance or visibility into IoT deployed in their environment, much less thought about deploying purpose-built IoT IDS to protect it, according to Laliberte.
Protivit advises organizations to realize that IoT must be managed and secured just like traditional IT, he said. In many instances, proper management of IoT can be an even bigger risk to the organization than traditional IT because of potential health and safety impacts if something goes wrong.
“Until organizations realize this and focus on IoT security, the demand for IoT IDS may not be great enough to fuel and fund the extensive research and development efforts needed to rapidly mature IoT IDS,” Laliberte said.
Some may argue that there has been a lot of research and work done to continuously improve AI-based IDS tools that are used in ecosystems with the IoT products in operation, Herold said. However, it is also true that IoT products are also being updated and new IoT devices and products are being introduced to the market continuously.
These are some widely documented problems and challenges for AI-based IoT IDS tools, according to Herold.
Will the autonomous actions taken for a false security alert result in harm to those using the ecosystem, digitally, physically or otherwise?
Will the AI-based IDS tool be able to consistently and accurately work in all types of network traffic situations?
Many IoT devices do not store data at all, so AI-based IDS tools that have analysis dependencies from data in memory and/or in storage may not be accurate.
The Future of AI-based IDS to Detect IoT Attacks?
Protivit is seeing major players making significant investments in the IoT security space. For instance, Microsoft is making major investments with its Azure Defender for IoT suite, according to Laliberte. This will help mature the space quickly, but businesses would need to recognize the need for IoT IDS and monitoring and then invest in the technology to continue to mature this technology.
“As standards further develop and emerge in the IoT space, it will become easier to develop and train AI/ML IoT IDS models,” he said. “I see this space continuing to evolve over the next few years, with a strong, mature product emerging in the next two years.”
About the Author
You May Also Like