AI Drives Discovery of New Materials
Researchers in Japan are using AI to make stronger metal alloys or discover new materials, revolutionizing the manufacturing process
December 19, 2023
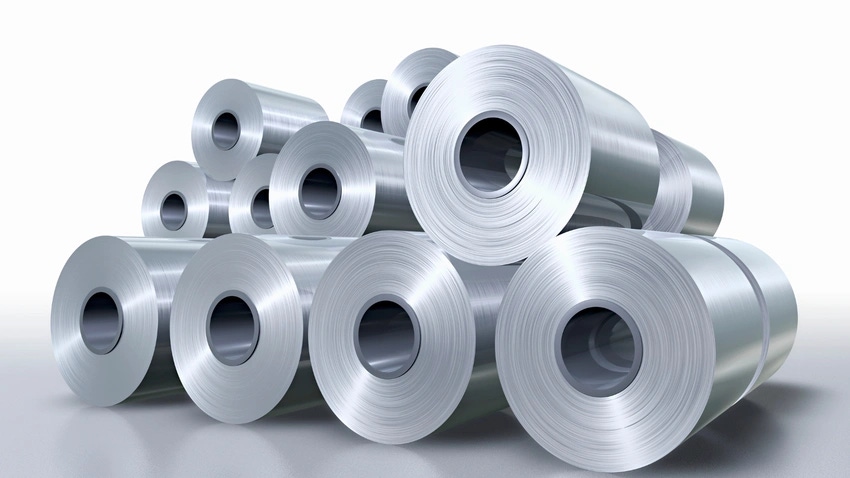
AI is revolutionizing the discovery of new or stronger materials that stand to transform the manufacturing industry.
Researchers in Japan have developed a novel two-step technique that enhances the strength of nickel-aluminum alloys – a material combining both metals widely used in the aircraft and other industries − at high temperatures, surpassing the traditional method of heating these metals.
The approach uses AI to pinpoint a variety of thermal aging schedules. “AI is useful in materials because you can virtualize experimentation, running thousands of experiments with a fair amount of predictive accuracy,” Jake Thiede, the COO of InFLOWS AI, who was not involved in the study, said in an interview.
“Materials have many different properties which you may be trying to balance, including the properties of the finished good and the performance under various conditions. An AI system is extremely helpful because Excel only goes so far when there are billions of different possible combinations and variable performance on the output side.”
Tougher Alloys
The new material technique showcases how machine learning is changing a scientific field that has traditionally relied on trial and error, according to scientists from Japan’s National Institute for Materials Science and Nagoya University.
To tackle the problem, the researchers had to examine how nickel-aluminum alloys are formed. To make these alloys stronger at high temperatures, controlling the size and amount of the phase that develops during thermal aging is essential.
There are many possible combinations of temperatures and times. For example, breaking the aging process into 10 parts with nine different temperatures can lead to about 3.5 billion combinations. Because of this, earlier attempts to find the best aging schedule mostly used constant temperatures.
The research team first used computer simulations instead of experiments to save time and money in evaluating these combinations. However, it was still not practical to simulate all 3.5 billion combinations.
Then, the team used an AI algorithm called Monte Carlo tree search (MCTS) to narrow these combinations to a smaller number of the best ones. They found 110 patterns using MCTS that were better than the usual constant temperature aging.
At first, these new patterns seemed complex and very different from the usual method. But after a closer look, the researchers understood them: Starting with a short period of high-temperature aging lets the γ´ particles grow to almost the right size, and then aging at a lower temperature for longer prevents them from getting too big while increasing their amount.
With this insight, they created a new two-step aging method: Start with a short high-temperature aging, followed by a long low-temperature aging. This method made Ni-Al alloys stronger at high temperatures than those made with any of the patterns found by the AI.
AI for New Materials
Designing new alloys is a complex task. The creators must decide on various factors, such as which elements to use, their amounts and the right combination of temperature and time for processing to get the best structure and features, Tanner Kirk, a materials design engineer at QuesTek, a materials consulting firm, said in an interview.
Researchers must ensure the alloys meet all essential properties, including strength, flexibility, weight, resistance to corrosion and wear, lifespan and cost. AI can search this vast space more efficiently than humans to find the best possible alloys.
Many scientists are now combining computer models that simulate material behavior, known as Integrated Computational Materials Engineering, or ICME, with AI optimization methods to explore vast ranges of materials for the best designs, Kirk said.
These AI-enhanced techniques allow designers to consider more variables simultaneously, often leading to better designs. Some AI methods, like Bayesian Optimization, can even recommend the next experiment to conduct, usually one that might reduce uncertainty in the model or increase the chances of finding a superior design.
Another increasingly popular way AI is used in materials design is through creating surrogate models. These models mimic the accuracy of detailed, first-principle models but need much less computing power. For instance, calculating the behavior of certain materials might typically take hours or days, but with these AI models, it can be done in just a few seconds. This speed-up is valuable in the design process, as it lets designers quickly evaluate many more potential designs.
AI models for predicting materials behavior are becoming more common, but they often do not include physics-based elements, making them less reliable for situations outside what they were trained on. These models' lack of physical principles can lead to unpredictable results. Adding physical understanding to AI models in materials science is a crucial area of research and is expected to boost their use by materials scientists and engineers.
Thiede said that companies are getting better at collecting the data needed to power material-selection systems, in which the characteristics of individual molecules are closely examined. When this level of analysis is possible, it opens up the opportunity to virtually test new materials that have never been used in the real world.
With a database of molecules and their properties, along with AI models that predict how an unknown molecule might behave in different conditions, it is possible to discover new materials, drugs and proteins, Thiede said. For instance, Insilico Medicine is using it to develop a drug named INS018_055 to treat idiopathic pulmonary fibrosis. NotCo is applying it to find unique proteins for plant-based milk. In materials science, it is being used to understand and create new ceramics, polymers and more, similar to how a ‘Ceramic Genome’ is being studied and understood.
One way to make AI models more understandable is through symbolic regression, Kirk said.
This technique produces standard analytical equations with terms that have real-world physical relevance. The equations are similar to the phenomenological ones that scientists and engineers have used for a long time. Because these equations are more familiar, they will likely be more widely accepted and used in the field.
“With the advent of large language models or LLMs that can write executable code, it is foreseeable that future AI models of materials can produce interpretable logic for models of even the most complex behavior,” Kirk added.
This article was first published on IoT World Today's sister site AI Business.
Read more about:
AsiaAbout the Author
You May Also Like