Indoor Climate Change: Understanding the Air We Breathe Through Data (Part 3)Indoor Climate Change: Understanding the Air We Breathe Through Data (Part 3)
November 9, 2021
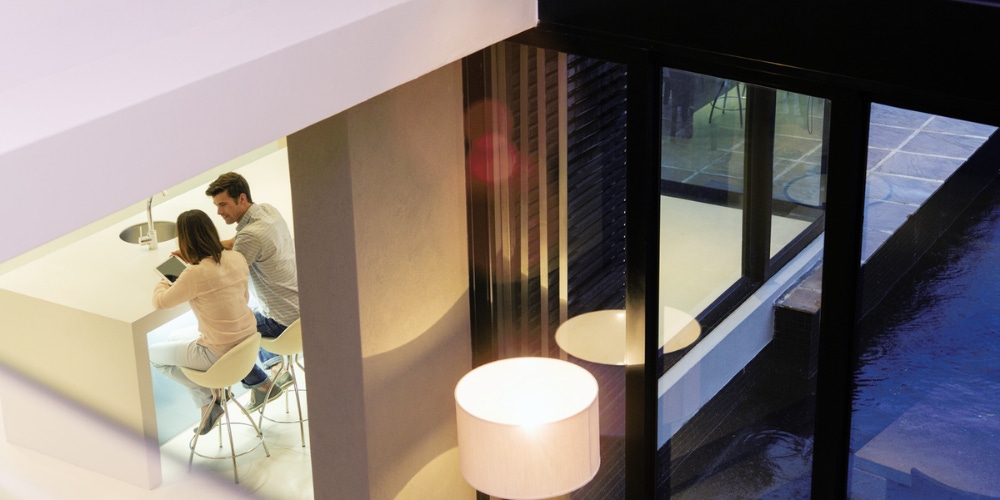
Sponsored Content
In Part 1 and Part 2 of this series, we discussed how we could glean significant insights from CO2 measurements using machine learning (ML). In this article, we will discuss specific CO2 measurements and the conclusions that have been drawn from them.
Figure 1: Events and associated CO2 levels over a few days in the author’s home
One additional advantage of machine learning algorithms is that they can automatically identify patterns and events from data when trained properly. Figure 1 illustrates the temperature and CO2 level variations in the author’s home and car during a few days of observation. From this figure, one can observe that opening windows leads to a drop in CO2 levels, while closing windows (in home or while driving) leads to an increase in CO2. Machine learning algorithms can be trained on such labeled examples, and will eventually learn to y identify such patterns to provide insights accordingly
In larger buildings, these insights can be used to save energy. Monitoring CO2 levels enables us to infer occupant activity (for example, rises in CO2 are tied to higher occupant activity or an increase in occupants). Understanding occupant’s patterns can help us manage energy consumption more effectively. If we can predict when a person will be in the building, the air can be recycled (needing heating or cooling) when energy prices are lower. This allows the heating ventilating and air conditioning (HVAC) system to coast through the day and save energy without needing to turn on when energy prices are high. This is a systems approach to HVAC and air quality problems.
To obtain greater insight into the potential impact of this approach, we took measurements using Infineon’s PAS CO2 sensors. The measurements were taken from hundreds of sensors every 5 minutes for many months. An explanation of the averaged daily, weekly and monthly data provides a sample of the value of the measurements, which you can see below.
Figure 2 shows the average CO2 levels that can occur in two rooms in one building every week averaged over a month-long period. We observe that CO2 levels peak on Monday in one room and peak on Tuesday is another, and then drop down over the rest of the week. This pattern shows that there are significantly higher CO2 levels earlier in the week, and implies higher use at those times, where tighter temperature control and more fresh air may be appreciated.
Figure 2: A one-week sample of the rise and fall of CO2 levels for two rooms in an office building.
With this type of data, building maintenance, operations, or an inspector can obtain insights into the effectiveness of the air circulating system. For example, a surprising finding from our data above suggests that there is CO2 build up on Sunday. Is this because ventilation systems are turned off, leading to higher CO2 levels earlier in the week? Could adding ventilation late Sunday improve user comfort on Monday?
Machine Learning for Occupancy Detection and CO2 Predictions
CO2 sensors can also be employed to predict the occupancy within a room. Figure 3 provides a more detailed set of measurements and occupancy interpretations. The top portion of the graph shows the CO2 levels in a room and the bottom is a ML algorithm predicting if at least one person is in the room or not–without requiring a motion or normal occupancy sensor.
Figure 3: Top figure shows CO2 levels in an office room, while the bottom shows the occupancy as a binary value (1 = occupant present, 0 = no occupant). Source: Open-source software.
Author’s Note: In the middle of the top graph, a straight-line spike exists, which is a best-guess prediction for positive Occupancy given missing data.
The algorithm predicts that a person will be in the room at a specific time. For an HVAC system, it is important to know when someone will come into a room and then, from that prediction, turn the ventilation off or on as required.
For individual rooms in an office building, this type of data can be used to create an optimal environment for occupants. With such predictive capabilities, we can make ventilation adjustments to the room, for example, to lower the CO2 level before the onset of a meeting in anticipation that several occupants may increase the CO2 level.
Understanding the air we breathe through data can have many positive impacts on human health and society. From the example dataset discussed here, we learned that the optimal level of CO2 indoors can alleviate negative health effects, such as drowsiness and poor concentration, leading to more productive employees. And, as we discussed in our previous article, large discrepancies between indoor and outdoor CO2 levels can be an indicator of airborne diseases. With CO2 measurements as a proxy and with further research, we can start identifying mitigation strategies to protect our communities. Although we are only just beginning to understand these measurements and their meanings at a global level, we have found that sensors can provide great insights into the air we breathe.
System designers and developers can learn more about the Infineon PAS CO2 at this link.
You May Also Like