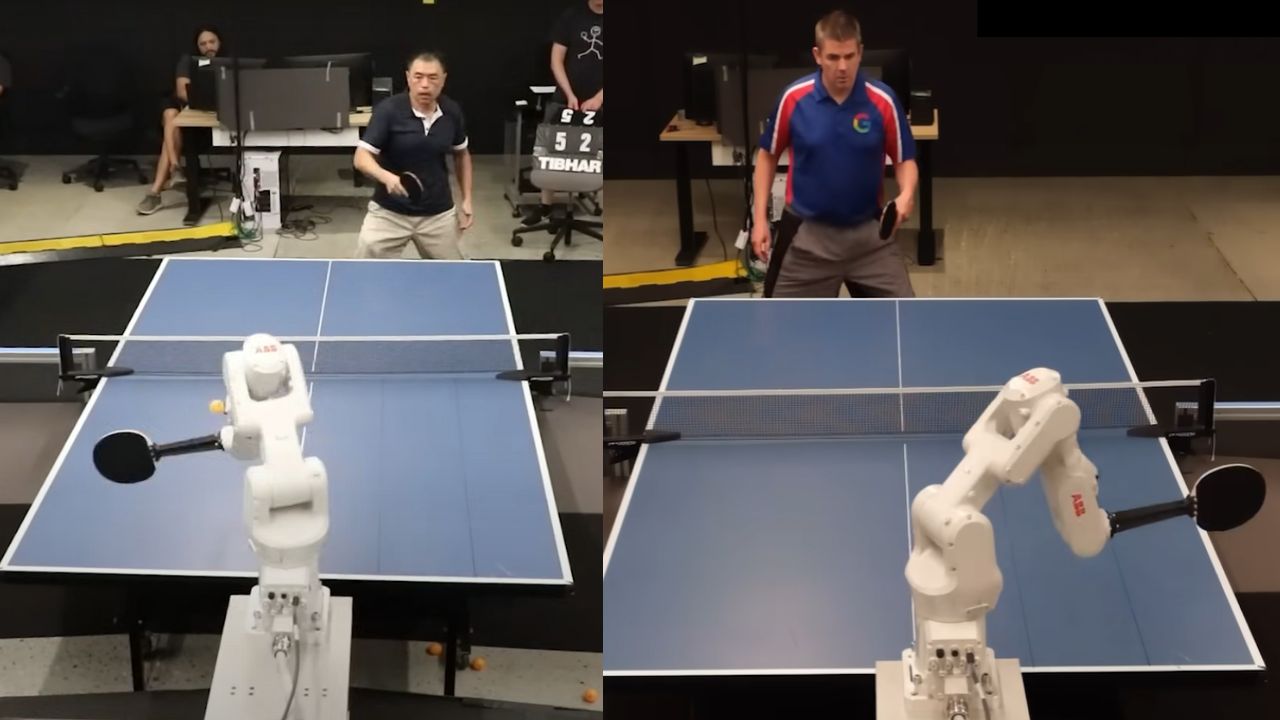
Google DeepMind researchers have developed an AI-powered robotic arm capable of playing table tennis at the performance level of amateur human players.
The robotic agent can adapt to an opponent’s actions in real-time, allowing for enjoyable table tennis games with human players.
The underlying AI agent was trained on a dataset of table tennis ball states, including position, speed and spin, which the system used to learn low-level skills, like forehand serving or backhand targeting.
The robot was initially trained in a simulated environment to allow it to master the real-world physics of table tennis matches accurately.
Upon real-life deployment, the robot applies its learned skills to play the game while collecting performance data. This data is then used to refine its skills in simulation, creating a continuous feedback loop.
Robotics researchers have long dreamt of developing systems capable of matching human-level speeds and performances.
Google DeepMind’s team said its latest effort “takes a step towards that goal.”
Compared to strategic games like Chess and Go, which robots mastered decades ago, sports like table tennis require speed, accuracy and adaptability.
The researchers said they turned their attention to table tennis because the sport presented a “valuable benchmark for advancing robotic capabilities,” including real-time precision and strategic decision-making and the ability to compete with a human opponent.
They began the development process by gathering a small dataset of human versus human gameplay to set initial conditions. The robot was then trained in a simulated environment using reinforcement learning and other advanced techniques.
Once trained, the robot’s policies were tested and refined on real hardware through a zero-shot deployment approach.
This iterative training-deployment cycle not only enhanced the robot’s performance but also adapted the complexity of play to match real-world conditions.
By continuously generating new training scenarios and incorporating them into the learning process, this hybrid simulation-real-world approach fosters a dynamic curriculum that drives ongoing improvement in the robot's skills.
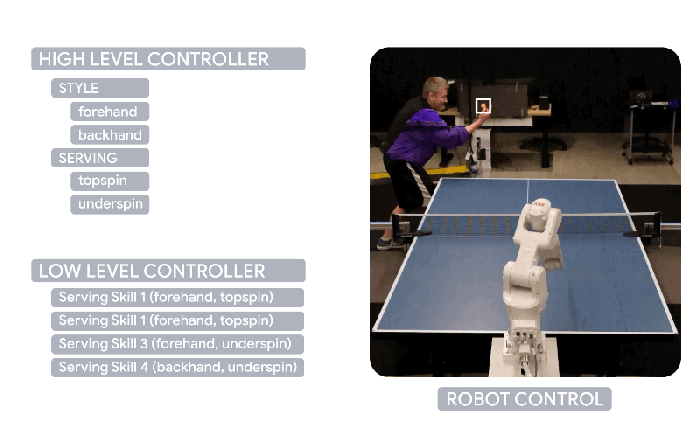
Credit: Google DeepMind
The robot lost all its matches against more advanced players, who were able to exploit weaknesses in the robot's policies
However, the robot won all its matches against beginners and 55% of games against intermediate players.
Google DeepMind said the results demonstrate “solidly amateur human-level performance.”
The robot did struggle to handle lobs and fastballs “due to [a] lack of data and hardware limitations.”
“Truly awesome to watch the robot play players of all levels and styles.” said Barney J. Reed, a professional table tennis coach. “Going in our aim was to have the robot be at an intermediate level. Amazingly it did just that, all the hard work paid off. I feel the robot exceeded even my expectations. It was a true honor and pleasure to be a part of this research.”
About the Author
You May Also Like