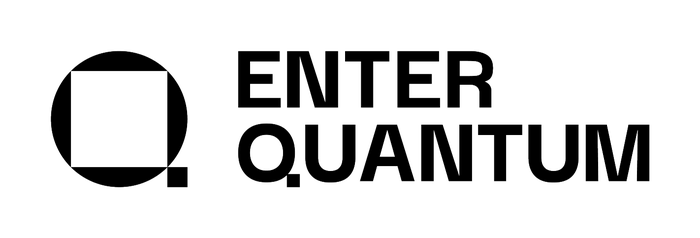
Connects decision-makers and solutions creators to what's next in quantum computing
Moody’s Taps Quantum to Predict Tropical Cyclone Intensity
Q&A with Moody’s
July 24, 2024
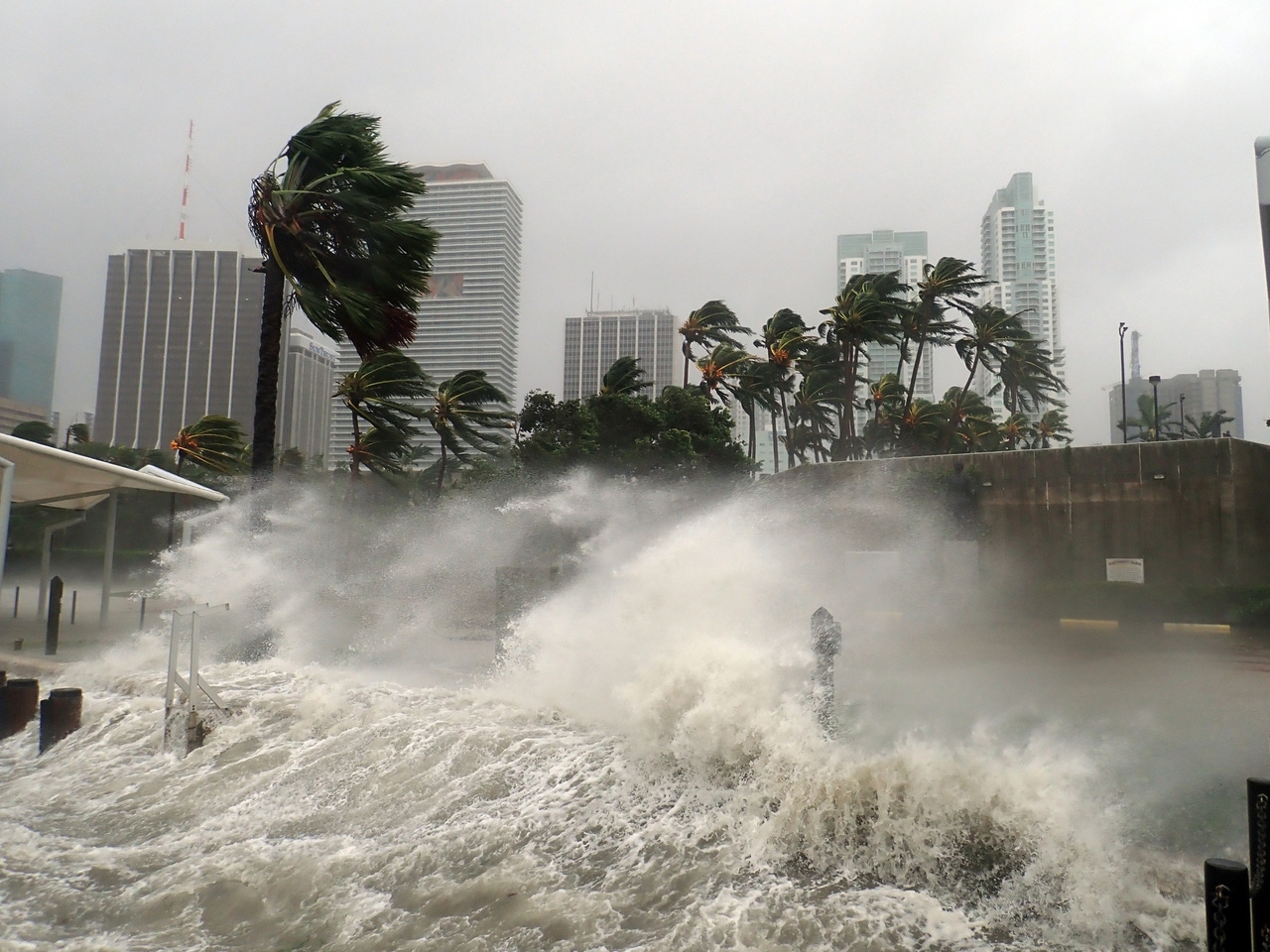
Risk assessment company Moody’s is experimenting with quantum computing to more accurately predict the intensity of tropical cyclones and improve the quality of its risk management services (RMS) tropical cyclone modeling suite.
The company’s RMS Hwind product helps insurers, brokers and capital markets understand the range of potential losses across multiple forecast scenarios, capturing the uncertainty of how a tropical cyclone’s path and intensity will evolve.
Moody’s is partnering with quantum computing company QuEra to apply reservoir computing (RC), a novel machine-learning algorithm particularly suited to quantum computers, to the problem.
In this Q&A, Moody’s quantum team director and team lead Carmen Recio and quantum computing engineer Ricardo Garcia explain the importance of the project.
Enter Quantum: Why is Moody’s exploring quantum computing?
Carmen Recio: Moody's is over 100 years old. It started as a rating agency and now we like to define ourselves as a global integrated risk assessment firm. We live in a new era where risks multiply and overlap. We call this the Era of Exponential Risk and our mission is to be the leading source of relevant insights on exponential risk. These risks are represented by mathematical models and we employ a lot of analytical techniques to extract information like advanced statistics and numerical analysis. We continuously assess whether we can benefit from using any new technology to get better insights or get the information that we need faster. We are exploring whether we can use quantum to solve the use cases that we solve today for our clients better or faster.
Why are you investigating tropical cyclones in particular?
Carmen Recio: This is part of our decision solutions marketplace that focuses on insurance solutions and analyzes the economic impact of events such as natural disasters. We have a whole portfolio of climate risk products, including one called Hwind, for real-time event preparation and response.
The Hwind team is not in charge of doing the forecasting. They consume the data from the meteorological agencies. But sometimes they have certain biases because these are rare events so data might not be available. The main goal of this project is to understand whether we can come up with a way to bias-correct the data that we get from the meteorological agencies.
Ricardo Garcia: More machine learning workflows are now being integrated into climate modeling. It is well known that the biggest application of machine learning hasn't been in the scientific community so far. We've seen it applied to text, audio and images but scientific applications are lagging.
That doesn't mean there aren’t a lot of academic-industrial groups out there trying to integrate machine learning workflows into climate modeling, and Moody’s is doing so. We are currently using classical machine learning to improve the forecast by bias correcting it. A lot of the state-of-the-art models used by operational forecasting balances are based on what are called dynamical models. These dynamical models try to explicitly model and resolve for all of the atmospheric and physical processes that can go on in the atmosphere. You can enumerate these processes, then you should be able to forecast the state of the atmosphere at future points in time.
These are run on classical supercomputers, but they suffer from these climate biases because having to be able to explicitly model for all these processes means we might overlook one of them. It is also very computationally costly to run. In the short and medium term, we can predict climate variables such as humidity, precipitation and temperature, but accounting for local and fine grid processes is computationally intense. If you want to look at the intrinsic characteristics, such as the track or intensity of a tropical cyclone, by looking at the eye of the hurricane or tropical cyclone, you need better resolution, you need more spatial resolution.
These models are not able to do that, so what happens is there is a mixture of these models, statistical or machine learning models coupled with coarser dynamic or climate models. This field is trying to get the best of both worlds and we are seeing machine learning being applied more and more in a hybrid context.
You're using reservoir computing, a machine-learning algorithm that works well on quantum computers. What is that and why does it suit this use case?
Ricardo Garcia: Classical reservoir computing is essentially a type of machine learning method that is well suited for these kinds of spatiotemporal problems. It stems from early works on recurrent networks, which were used for sequence modeling, and they have been applied to things like chaotic time series predictions and studying the variability of climate or weather models. So we know that reservoir computing classically has salient characteristics for this kind of task.
By collaborating with QuEra, which is providing the quantum hardware for this project, we found out that one of the most well-suited parts of machine learning algorithms to run on quantum computers was the quantum reservoir algorithm, which was also suited for this task. By swapping the black box of classical reservoir computing to a quantum processor, in this case by QuEra's processor, we can investigate whether these types of algorithms work better using quantum computing.
Is Moody’s investigating any other quantum computing use cases?
Carmen Recio: We have a big backlog of use cases and we’re trying to prioritize the goals that we think are more near-term. We're working in the three main areas.
We have optimization problems, simulation or stochastic modeling, and quantum machine learning. In machine learning, in addition to the tropical cyclone project, we have worked with Rigetti Computing on enhancing the task of predicting recessions.
There's a whole idea of whether quantum machine learning or even quantum-inspired techniques can give us a white-box approach versus the black-box approach that we have with certain AI algorithms. This is a very big problem for applying AI in finance because it's a highly regulated field.
The big use case in optimization is portfolio optimization. We need to impose certain constraints that restrict the search space where you can find your optimal solution because of regulations. That makes it harder for the algorithm to find it. In some cases, we need more complex models to more accurately represent reality or we want to do optimization dynamically, meaning our algorithms struggle. We may also want to consider risk metrics that are more complex than the naive ones some models use. We consider all these optimization problems as a single block, because the techniques that we have for one of the problems may apply to others as well.
We're also considering benchmarking different quantum solvers for optimization problems. Several companies provide solutions for quantum optimization. However, we don’t want to believe anything a priori, we want to test it ourselves. We plan to publish a white paper on that after the summer.
A longer-term area is simulation, for example for pricing derivatives, risk analysis and anything else where we could use, for example, Monte Carlo simulation. Also, we are looking at problems with partial differential equation systems that are high dimensional. We are thinking about how to better solve these systems with techniques that reduce the complexity. An example is flood inundation mapping, also related to climate risk. We are going to collaborate on this with ETH Zurich as part of their Masters program in quantum engineering.
About the Author
You May Also Like