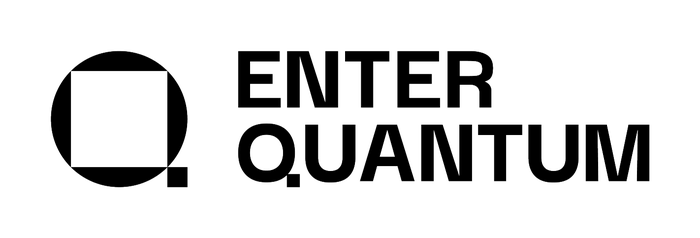
Connects decision-makers and solutions creators to what's next in quantum computing
Quantum Accelerates Fraud Detection Machine Learning
IBM panel details how quantum supports classical machine learning
.jpg?width=1280&auto=webp&quality=95&format=jpg&disable=upscale)
The financial services industry is often promoted as one of the earliest sectors to benefit from quantum machine learning, and an important use case is fraud detection. But how could it work to speed up the calculations classical computers struggle with and how can it help fraud detection operate in real time?
Machine learning on classical computers has significantly improved financial fraud detection capability. Speaking on the “Qubits and neurons to turbocharge the world of finance” panel at an IBM quantum and AI event last week, IBM quantum applications researcher Christa Zoufal explained that quantum computers can select the features that best train the machine learning model.
“In the financial industry, we have quite a lot of equipment and problems that have a very complex structure underneath, and with this complex structure it can get hard for classical algorithms to solve them,” she said.
“If you want to do fraud detection, for example, you can have a lot of data with features that represent the transactions. You don't just want to put thousands of features into your models because that would make the training very difficult. You want to collect a few very good ones to make the problem simpler to train faster, and to make it more understandable.”
Feature selection is a difficult problem and the number of solutions in the problem scales exponentially with the number of features that need to be considered. Most classical machine learning algorithms can, at most, look at correlations between two features, and even if there are 1,000 features, classical algorithms will look at correlations between one pair of these features at a time.
“What you can do with quantum machine learning in this particular context is run the algorithm using the quantum circuit and look at all of the correlations at the same time. In some instances, we have already found that with this quantum algorithm we can get a better picture with very simple models,” said Zoufal.
Zoufal said that although this is an early study, it shows that feature selection can be performed better on a quantum computer. Those selected features can be passed into classical machine learning algorithms to what consequences changing the value of these features has on detecting potential fraud.
She added that IBM’s experiment found a measure of how much information can be represented by a quantum neural network and how much by a classical neural network.
“We have some indications that the quantum neural networks can present more information with the same set of parameters than a comparable classical neural network,” Zoufal said.
“The take-home message is there are various aspects of quantum computing that can give us benefit in the quantum machine learning field. Now we need to identify the things that are difficult for classical computers and see where the quantum computers can get us.”
About the Author
You May Also Like