Setting the Stage for Industrial Data Science SuccessSetting the Stage for Industrial Data Science Success
Industrial data science projects demand organizational-level skills as well as leadership and support.
April 1, 2020
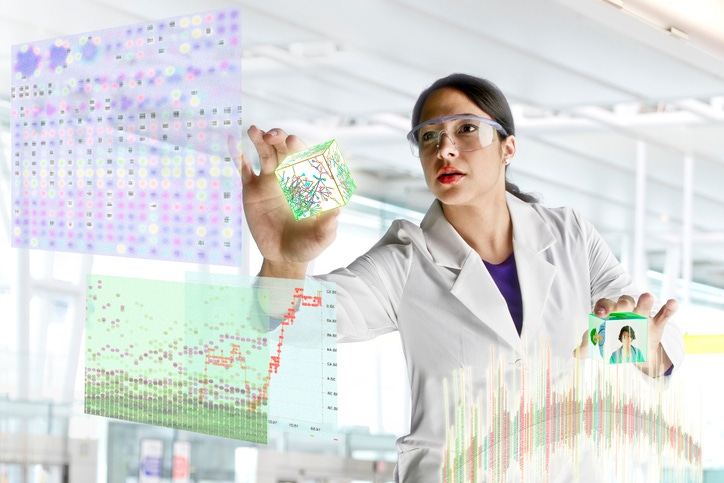
At some point, most successful industrial Internet of Things (IoT) initiatives become data science projects. Connected sensors on machines and equipment, tools, pallets and manufactured items generate volumes of data points.
But the prospect of business or operational success hinges not on data collection alone, but on a broad range of skills extending across the organization. It also requires using that data to fuel transformation. That might include achieving new operational benchmarks or, “in today’s world, creating resilience and flexibility,” said Steve Pillsbury, principal at PwC Consulting.
[IoT World is North America’s largest IoT event where strategists, technologists and implementers connect, putting IoT, AI, 5G and edge into action across industry verticals. Book your ticket now.]
Even before the emergence of the COVID-19 pandemic, driving an industrial data science metamorphosis was daunting for many organizations. Data science experts were in short supply, and hiring competition was fierce. While many industrial organizations have launched digital innovation programs, few have reach the desired return on investment. From 2016 to 2018, digital projects at nearly 80% of industrial companies failed to achieve the expected level of financial returns, according to Accenture research.
Preparing the Organization
One reason industrial organizations struggle with industrial data science is lack of planning. “Most [industrial] companies now are, at a minimum, in the pilot and test phase and, in many cases, are in the application and adoption stage,” Pillsbury said.
One factor holding them back from reaching scale and value is lack of “know-how,” Pillsbury added. “That doesn’t mean that they have, necessarily, the wrong [technical] skills or not enough of the right skills. It also means they haven’t really prepared the organization to apply the types of skills that are needed.” Industrial organizations must educate their entire staff on “what the art of the possible is, and how to embrace it,” he said. That is, organizations need to be skilled at cultural transformation as well.
PwC’s Digital IQ research found that, in various sectors, organizations with the most successful digital programs built them around their people and culture. Dubbing digital organizations “transcenders,” PwC found they prioritized educating employees and creating a resilient culture.
Within an organization, however, data maturity of employees and departments can vary considerably, observed Murali Raj, chief information officer at HIL, a building supply manufacturer. In developing a transformation plan, account for that variability and build a broad-based data foundation, Raj recommended. When HIL deployed predictive maintenance, the company built a foundation to make the most of data. “Rather than focusing on a small manufacturing line or a couple of machines for predictive maintenance, we focused on creating a digital, connected shop floor backbone,” Raj said.
Human-Centered Design Applied to Processes
Successful industrial organizations win buy-in from senior and junior-level employees for digital and data-science initiatives.
Many organizations piloting such projects dedicate a team to digital leadership and best practices. Such “‘Center of Excellence’–type people, tend to understand technology and data science really well, and have a general idea of the value drivers and the problem statements they’re trying to address,” Pillsbury said. But when those experts build digitally enabled tools for others to use, “they find that people generally don’t like them,” Pillsbury said. End users often “don’t like design, the way [a piece of technology] works, how they are supposed to interface with it, or what to do with new information,” he added.
Organizations can gain employee support for data-driven digital tools by soliciting feedback during design, recommended Pillsbury. Digital and data leaders can also deploy human-centered design concepts to internal processes, studying the context of a given problem as well as employees’ pain points before devising digital tools or workflows to address them.
Identifying Individuals to Lead Transformation
While cultural transformation and education are vital, there’s no substitute for data science leadership. While scores of individuals have added terms like “data science,” “artificial intelligence,” “machine learning” to their résumés, only one out of four candidates are experts, according to Umesh Ramakrishnan, office of the CEO at executive search firm Kingsley Gate Partners. “By definition, a lot of these [artificial intelligence] skill sets are new,” Ramakrishnan said. It should be a red flag “if somebody tells you that they have 25 years of data science expertise.”
When interviewing potential data science leaders, it should take 20–30 minutes per person to identify whether they have significant knowledge, according to Ramakrishnan. “A lot of these folks have had a foundation in either science or engineering that’s related to artificial intelligence, machine learning or data science,” Ramakrishnan said. “People who have worked on neural networks or deep learning in the past and have continued to keep their edge sharp by either on-the-job experience or through additional education are the ones that we see entering mid- to senior-level positions.”
Data science experts who can share their expertise with others and induce change across the organization are most valuable. But screening candidates for strategic skills is significantly more challenging than identifying discrete technological expertise, Ramakrishnan said. “The technology transformation inside an industry is far less difficult than the cultural transformation of that company, especially if it’s a legacy company,” he said.
Industrial organizations with successful digital programs tend to have a shared vision across upper- and middle-management as well as the ability to “align talent pools and technology assets across key business functions,” according to Accenture. Data science leaders can help instill traits, but they must excel at communication and persuasion. “You have to have the ability to show people why it’s advantageous for a person on the shop floor to, say, use an iPad in a work context rather than a clipboard,” Ramakrishnan said. That person should also be able to explain the value of technology to a worker as well as the value to the company. “The ability to tie an individual’s objectives to the mission of the company is a leadership attribute that is very rarely seen in executives.”
Terms like “champion” or “evangelist” don’t do justice to this capability in Ramakrishnan’s opinion. “Those terms paper over the sophisticated leadership attributes that are required,” he said. Organizations moving proof-of-concept industrial IoT projects toward broader digital transformation initiatives need more than industrial data science experts who are articulate. “Simply being a good speaker can make you a good evangelist,” Ramakrishnan concluded. “But if you have no way of translating your evangelizing into actual execution, then all you are is a preacher, and you don’t need that in the business.”
About the Author
You May Also Like