A Guide to Using AI for IoT ApplicationsA Guide to Using AI for IoT Applications
Deploying AI for IoT projects has gathered momentum as the infrastructure for collecting and processing data matures.
March 12, 2020
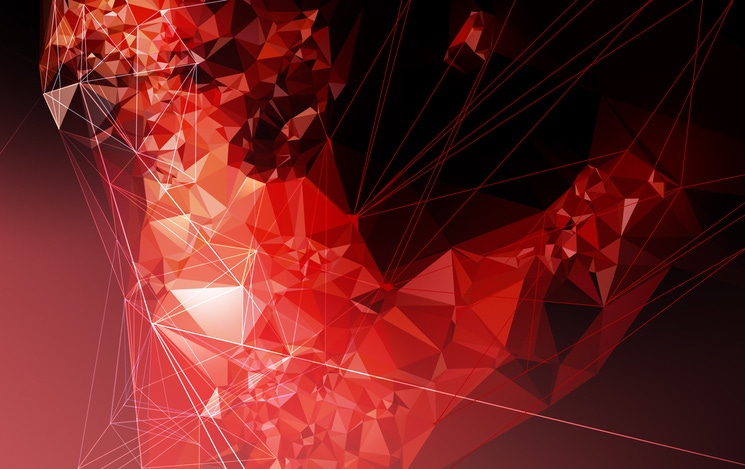
Despite the often-fuzzy definition of the term, artificial intelligence is looking like an eventual necessity for enterprise and industrial businesses — especially those with IoT deployments.
Machine learning and computer vision have already gained ground in many industries, and data science has become a focus for many organizations.
In an Internet of Things (IoT) context, artificial intelligence (AI) has become a prerequisite for dealing with the data volume sensors produce, according to Richard Soley, executive director of the Industrial Internet Consortium. “Anything that’s generating large amounts of data is going to use AI because that’s the only way that you can possibly do it,” he said.
The number of IoT projects that have created enormous amounts of data is steadily increasing. The Industrial Internet Consortium, for instance, has a testbed dedicated to deep learning that analyzes some 35,000 data points per minute. “They’ve sensorized an entire building outside of Tokyo,” Soley said. “It’s generating 300 terabytes of data per day.”
[IoT World is North America’s largest IoT event where strategists, technologists and implementers connect, putting IoT, AI, 5G and edge into action across industry verticals. Book your ticket now.]
Similar stories are increasingly common for organizations with mature IoT projects. A single oil well, for instance, generates some 10 TB of data per day, according to IHS Markit. IDC projects that by 2025, there will be 41.6 billion Internet of Things devices generating 79.4 ZB worth of data, which would equal 79.4 trillion GB.
As a result of the data flood, nearly two-thirds of organizations with IoT deployments also have an active big data analytics program, while more than half have a corresponding AI initiative, according to PwC.
A growing number of executives are also convinced of AI’s long-term potential. Today, 19% of executives believe AI is most important to their company’s strategy today, while one-quarter viewed IoT as a cornerstone technology, according to PwC research data shared with media. Three years from now, however, 42% of those executives projected AI to be the most vital technology for their company’s strategy, while the corresponding figure for IoT was 21%.
Making Artificial Intelligence a Long-Term Growth Driver
Organizations that resist deploying and scaling a variety of digital technologies risk becoming progressively more ill-equipped to operate in an increasingly volatile world, according to Mark Hermans, managing director at PwC. “The pace of change will only accelerate. The challenge is not whether to embrace productivity-boosting innovation, but how to make it work effectively and affordably,” Hermans said. “It is a matter of rapidly experimenting, learning, upskilling, reinventing and scaling in well-defined, agile methods while continuing to serve customers.”
Artificial intelligence remains at an early phase of adoption, and a minority of organizations have made AI a top investment priority. Those that have, however, tend to be rewarded for their efforts.
An EY survey found that while investment in AI trailed cloud computing, advanced analytics and the Internet of Things in terms of priority, 93% of organizations with mature AI projects saw positive results related to cost savings, efficiency or productivity.
Similarly, organizations that implement IoT and AI technologies in tandem are more likely to make routine data-driven decisions and to have more efficient operations and higher employee productivity than their peers, according to an IDC study.
“The data is out there abundantly showing that companies leveraging data are showing greater returns,” said Daniel Newman, principal analyst at Futurum Research.
But there’s a caveat. Achieving long-term success in using AI for IoT takes a long-term focus as well as experience. PwC research suggests that companies that excel at digital transformation are four times more likely to have at least 10 years of experience with their initiative.
While it is wise to consider the payback period and return on investment related to the AI initiative, the ultimate goal is to create a sort of feedback loop in which a project’s financial gains can be reinvested. “The returns will come in phases and will increase as the models become more tuned to the business,” Newman said. “Models will need to be developed, tested, refined and updated on an almost continuous basis.”
Be Realistic, Focus on Foundation for AI, IoT
It can be tempting to aim to create an advanced artificial intelligence initiative by choosing the most-advanced algorithms or the latest chip. A better indicator of future success, however, is the degree of investment in foundational infrastructure, including operating models, talent and training.
Another theme to consider is the type and quality of data involved. “Less is more with a lot of data projects. Isolate variables and optimize motions and then scale up,” Newman said.
It is also vital to ensure data is sufficiently applicable to potential data science models. “This is an area to focus on before over-investing in developing models,” Newman said. “The model with the best data will evolve into a powerful analytics tool as more data is fed to it.”
Work to Make Models Robust
While the availability of large data sets and cloud computing has propelled machine-learning advances, the data has to be accurate in the first place. In an IoT context, that means ensuring that instruments and sensors are accurately calibrated and that input data isn’t compromised, intentionally or unintentionally. “Garbage in, garbage out is true in IoT as much as anything else,” Newman said.
Ensuring machine learning algorithms function as intended is an important consideration. “It’s one of the biggest problems I worry about in the future era,” said Zulfikar Ramzan, chief technology officer at RSA. “People are relying more and more on sophisticated machine learning algorithms without really understanding how they work. In many cases, the algorithms are black boxes for them.”
An organization planning to use legions of IoT sensors in a machine learning model should proceed with caution. “Will you be able to calibrate hundreds of thousands of sensors to make sure they are accurate? The answer is ‘no,’” said Aleksander Poniewierski, global IoT Leader and partner at EY. “And even if they are accurate today, they will drift over time. If you build an AI model on top of improperly calibrated sensors, you ultimately risk having the model make a very bad decision.”
The difficulty of ensuring the integrity of exploding volumes of data and complex algorithms “create a layer of obfuscation and make it very difficult to identify that something has gone awry until it is maybe too late,” Ramzan said. “Even small amounts of noise can cause large disruptions in some machine learning models.”
Organizations should work to address biases or software bugs that can cause inaccurate data collection. Doing so is not always an easy task, Ramzan said. “When you consider all the real-world complexities of making machine learning operational, trying to get around these issues becomes very thorny in many cases.”
While the use of AI for IoT may be an essential tool for the future of business as well as for a growing number of IoT projects, the subject demands considerable planning, Ramzan stressed. “When you look at real-world machine learning systems, for instance, they often have a lot of messy parts, and getting these things to work operationally is far more complex than most people fully realize.”
About the Author
You May Also Like