Digital Twins in Health Already Helping in Disease Treatment
Digital twins in health care have matured to replicate even rare diseases such as heart conditions or COVID-19-related impact. Are these digital replicas ready for prime time?
March 11, 2021
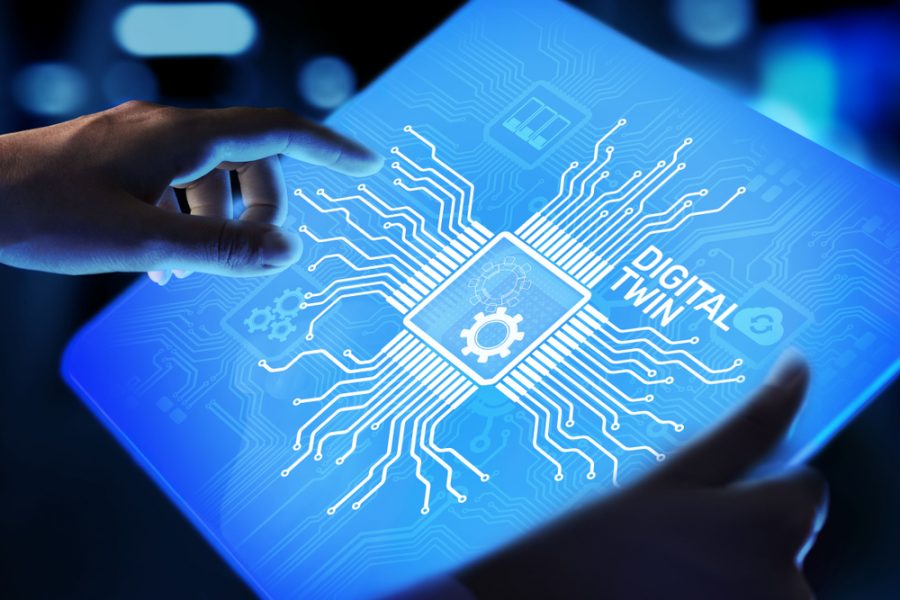
Digital twins in health care have developed to provide replicas of various health conditions, such as heart disease or even COVID-19 lung impact.
New developments have enabled digital twins in health to provide what-if problem solving for rare health conditions without having deleterious effects on patients.
Digital twins do pose potential big data challenges, but practitioners are working toward more targeted digital twin models.
Digital twins in health care have begun to help practitioners understand patient conditions, even novel ones such as COVID-19’s impact on the lungs.
A digital twin is a virtual representation of a physical object or system across its life cycle. It uses real-time data and other sources to enable learning and dynamic recalibration for improved decision-making as processes unfold. This highly complex digital model is a counterpart, or twin, of a physical thing, such as a car, a piece of equipment or a person.
Previously, digital twin technology was the province of product design, helping improve products before they were manufactured in the real world. But today digital twin technology has made its way into personalized care.
But today, digital twin technology is gathering momentum in health care diagnosis. Digital twins are upending traditional health care by modeling conditions and attributes of a particular patient using machine learning data aggregated from a variety of patients. Digital twins have now helped practitioners understand a variety of diseases, from heart anomalies to the impact of COVID-19.
“Just as this year has driven data collection, this has everything to do with digital twins. Biometrics have been accelerants, as well as a shifting regulatory environment,” said Kaleido Insights analyst Jessica Groopman.
Data suggests that digital twins in health is on a growth trajectory.
The global digital twin market was projected to be worth $5.1 billion in 2020 and $115.1 billion by 2035, growing at an estimated compound annual growth rate of 23%, according to ResearchandMarkets. Further, for companies implementing IoT projects, 18% have already implemented digital twins, and another 70% plan to implement within 12 months, according to a Gartner survey released in October 2020.
Digital Twin Technology in Health Provides Key What-If Analysis
For health care domains such as cardiology, digital twin technology has been a boon to better treat congenital heart defects.
“If we can understand how it works in the normal configuration, why can’t we understand how it would work in reverse configuration?” said Steve Levine, founder of the Living Heart Project at Dassault Systèmes.
“If we could take the normal heart and change it the way nature would change it, the changes that happen to the human body are not unpredictable.”
With digital twin technology, the project can replicate rare heart conditions, such as valves that are reversed from those of a healthy heart, and then, virtually, test certain treatments on that replica before administering anything to a patient.
“We can guide the treatment and base it on testing to see what will work best on the virtual patient before they actually try it on the real patient,” Levine said.
Solving Patient Data Challenges With Digital Twins
One critique of digital twins, and machine learning for health care, has been that it’s labor-intensive to train the data models with enough data to understand health conditions such as cancer, diabetes and heart disease. In the early days of developing these models, many health care professionals tried to feed the models with as much data as possible.
Traditionally, these methods have been computationally intensive, but they have progressed.
Today the goal is not to feed models as much data as possibl, but to provide targeted data that fuels “precision models.” This enables digital twins to solve the problem at hand.
This approach has allowed the Living Heart Project to develop targeted digital twins “templates” of healthy hearts as well as digital twins for hearts affected by certain conditions.
“The smaller you divide the data, the more coherent it’s likely to be, and then you can understand it,” Levine said. “It takes more discipline to do it piece by piece versus trying to solve the whole problem all in one go.”
Another key concern is how to secure personalized patient data. Digital twins can help in this regard by masking attributes.
The digital twin project focuses on aggregating patient data to remove individual attributes. Data is shared on an open source platform, which enables myriad researchers to access the data but also protects patient identity.